What is Data Science?
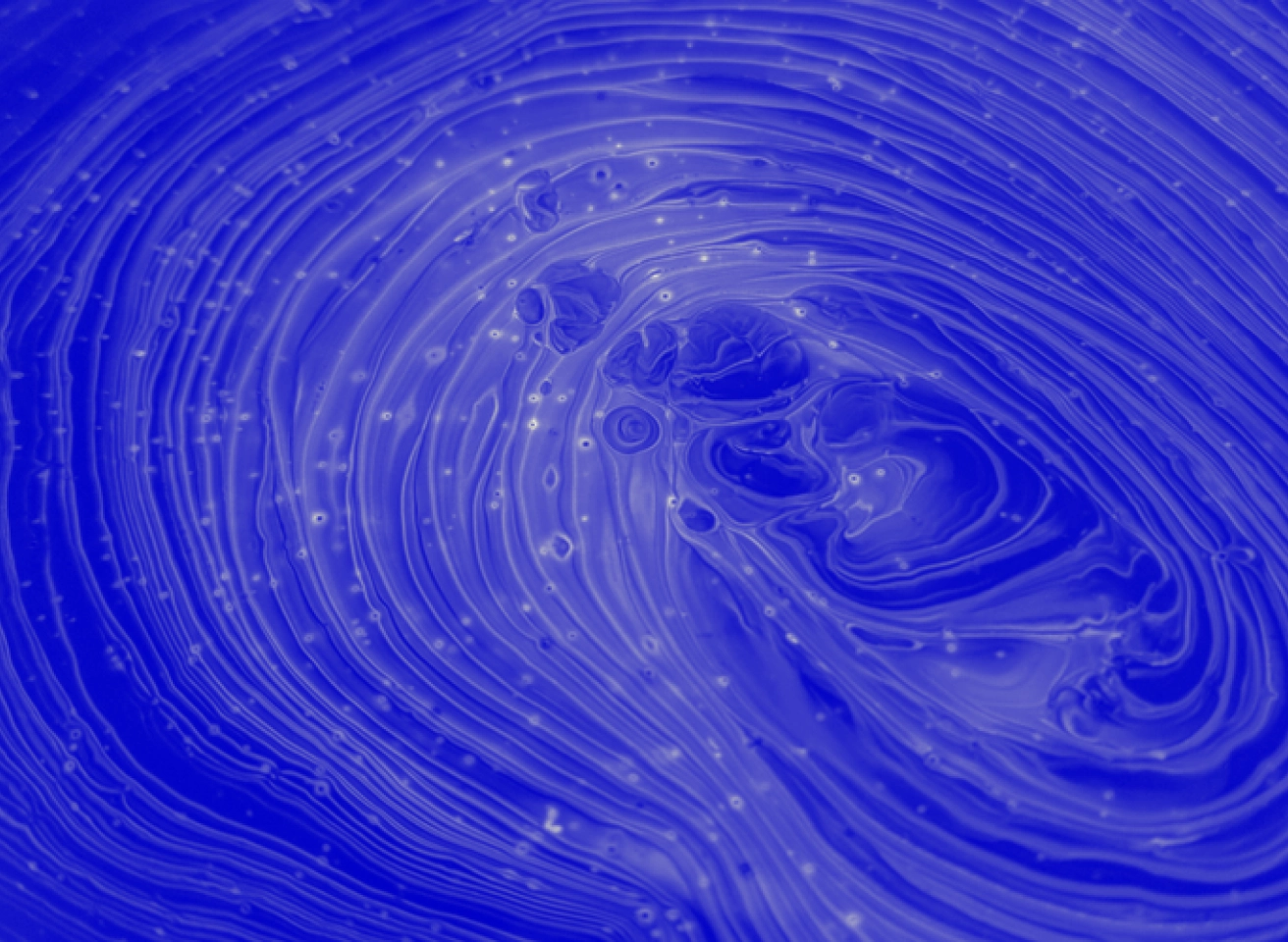
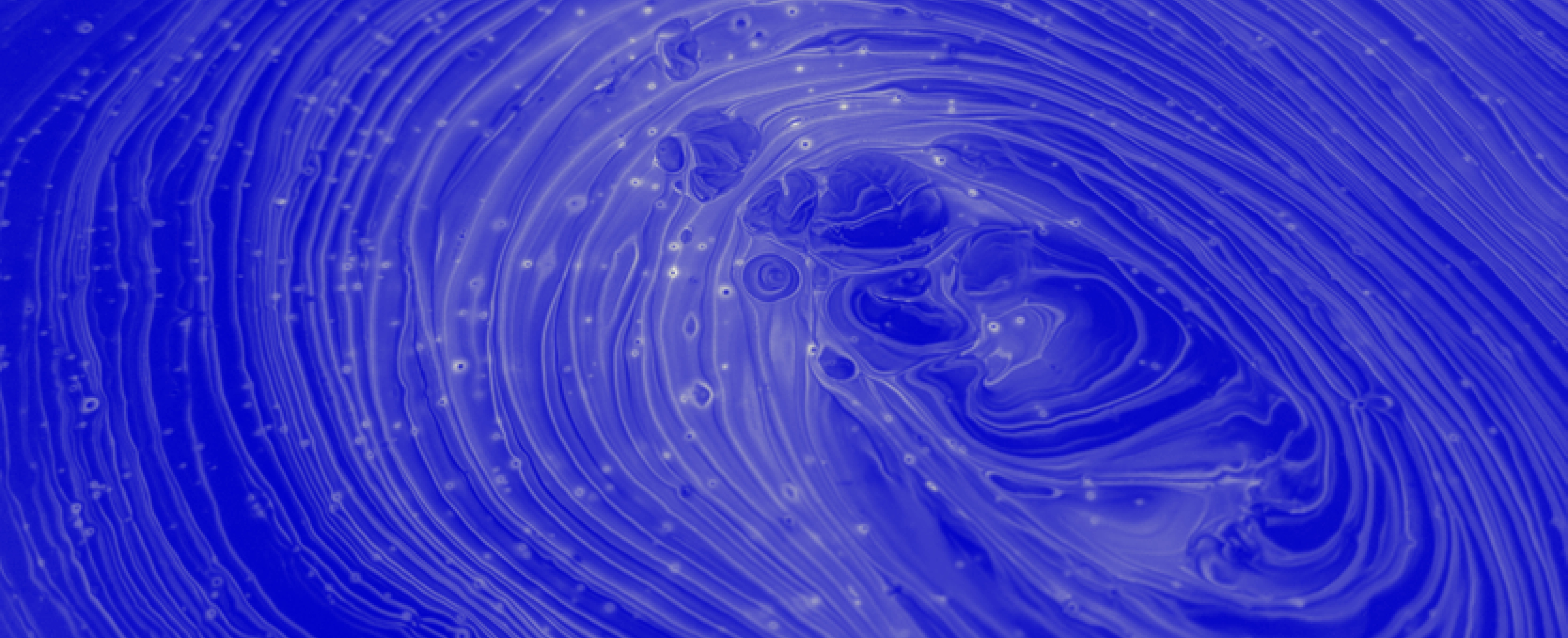
In recent years, data science has become increasingly prominent in the common consciousness. Since 2010, its popularity as a field has exploded. Between 2010 and 2012, the number of data scientist job postings increased by 15 000%. In terms of education, there are now academic programs that train specialists in data science. You can even complete a PhD degree in this field of study. Dozens of conferences are held annually on the topics of data science, big data and AI. There are several contributing factors to the growing level of interest in this field, namely: 1. The need to analyze a growing volume of data collected by corporations and governments 2. Price reductions in computational hardware 3. Improvements in computational software 4. The emergence of new data science methods. With the increasing popularity of social networks, online services discovered the unlimited potential for monetization to be unlocked through (a) developing new products and (b) having greater information and data insights than their competitors. Big companies started to form teams of people responsible for analyzing collected data.
What is Data Science?
- Data Science is the study of data and its properties. It is focused on discovering the question from data to ask unlike just asking it.
- Data Science is a multi-disciplinary field of study, which combines domain expertise, programming skills, and knowledge of math and statistics to extract meaningful insights from data.
- Models in Data Science are not static; they are constantly tested, updated and improved in time. Thus, it becomes possible to obtain actionable information from data and not just historical facts.
Data Science tasks require knowledge and experience in different areas. Collection, analysis, interpretation, presentation and organization of data require a rich background in statistics, linear algebra and calculus. Experience in computer science and software programming is necessary for data manipulation and processing. Knowledge in domain expertise (business, philology, medicine, biology, and other related areas) provides insight into (a) the problems which require solutions and (b) the kind of data that exists in the domain. In addition, written and verbal communication skills are in high demand in Data Science. The probability of finding an expert in all aforementioned areas is close to the likelihood of finding a unicorn. Therefore, the most successful strategy is to build a Data Science team of specialists in different areas.
An organization with a high level of digitalization, and a skilled Data Science team can provide the main Data Science activities: description, discovering, prediction and advice. These activities are listed according to the increasing complexity of their implementation. At the first stage, a Data Science team uses basic analytic functions and refines raw data to describe data. For example, they could start with a description of customer distribution with respect to location. At the next stage, it is possible to discover hidden relationships or patterns in the data. This, in turn, allows the team to find possible groups of customers who purchase similarly. Then, past observations can be used to predict future observations. At this stage, the case study may center around, for example, predicting the products that certain customer groups are more likely to purchase. The final (and most challenging stage) involves defining possible decisions, optimizing over those decisions, and recommending the decision that gives the best outcome. Each of these stages can improve an organization's activity, but the greatest benefits are gained in the Predict and Advise stages.
The benefits of data science depend on the industry in which they are employed, and their goal(s). With this being said, the common advantages of enlisting data science for most organizations include: (a) improvement in operational efficiency and workflow, and (b) performance improvement in data-driven decision-making. Data Science continues to evolve rapidly, touching the most pivotal aspects of our lives: healthcare, financial trading, travel, energy management, social media, e-commerce, human resources, and fraud detection (to mention a few examples). The coming years will bring a deeper integration of Data Science into most domains of business, science and daily life.
Interesting For You
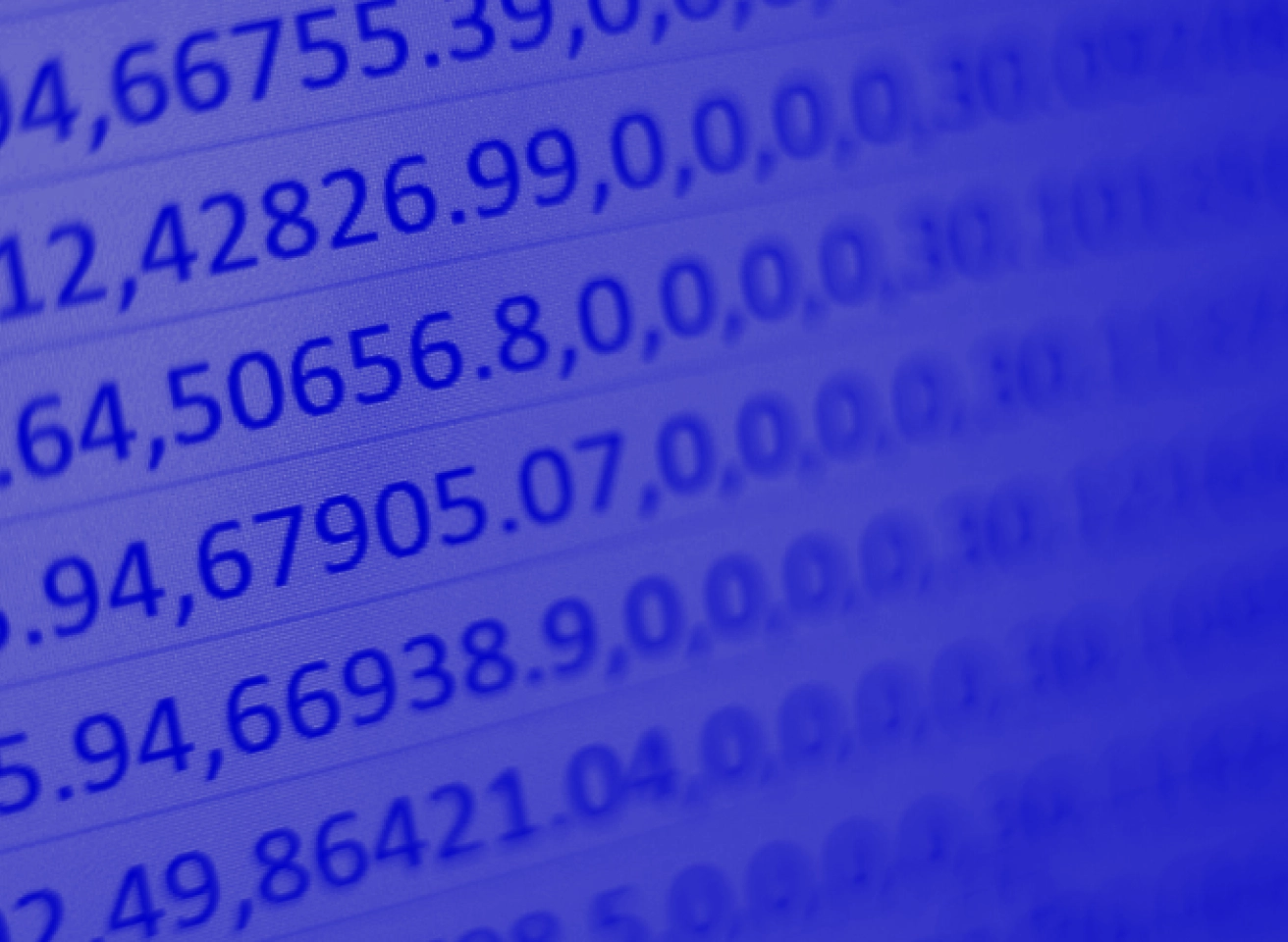
A Brief History of Data Science
Data science, AI, and Big Data have been the biggest buzzwords of the technological world over recent years. But even though there’s a lot of marketing fluff involved, these technologies do make a real difference in highly complex industries like healthcare, financial trading, travel, energy management, social media, fraud detection, image and speech recognition, etc. With the digitalization of the world economy and virtually every aspect of life, data has become the new oil (a term coined by Clive Humby). Subsequently, data science has become the sexiest job of the 21st century. But that’s really cutting a long story too short. Let’s look at the development of data science in more detail.
Read article
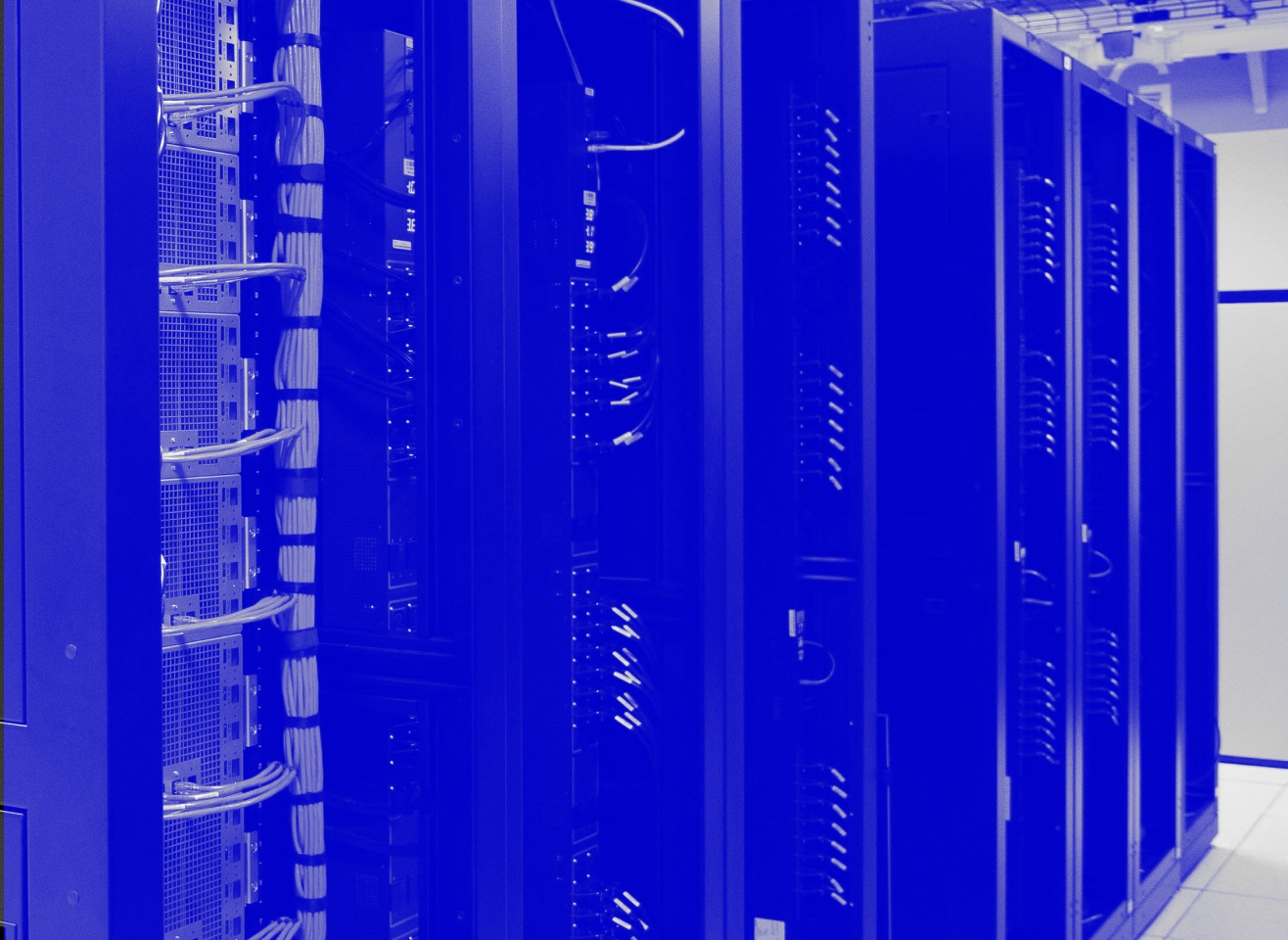
How Can Data Science Help My Organization?
Nowadays, there is a tendency to hire data scientists or even form data science groups in companies. This does not only apply to specific activity sectors or large organizations. Small and midsize businesses are more frequently involving data scientists, in order to get actionable insights from collected information. So, how does data help to run and grow everyday businesses? There are several areas where collected data and the insights drawn from that data can have a significant impact on business.
Read article
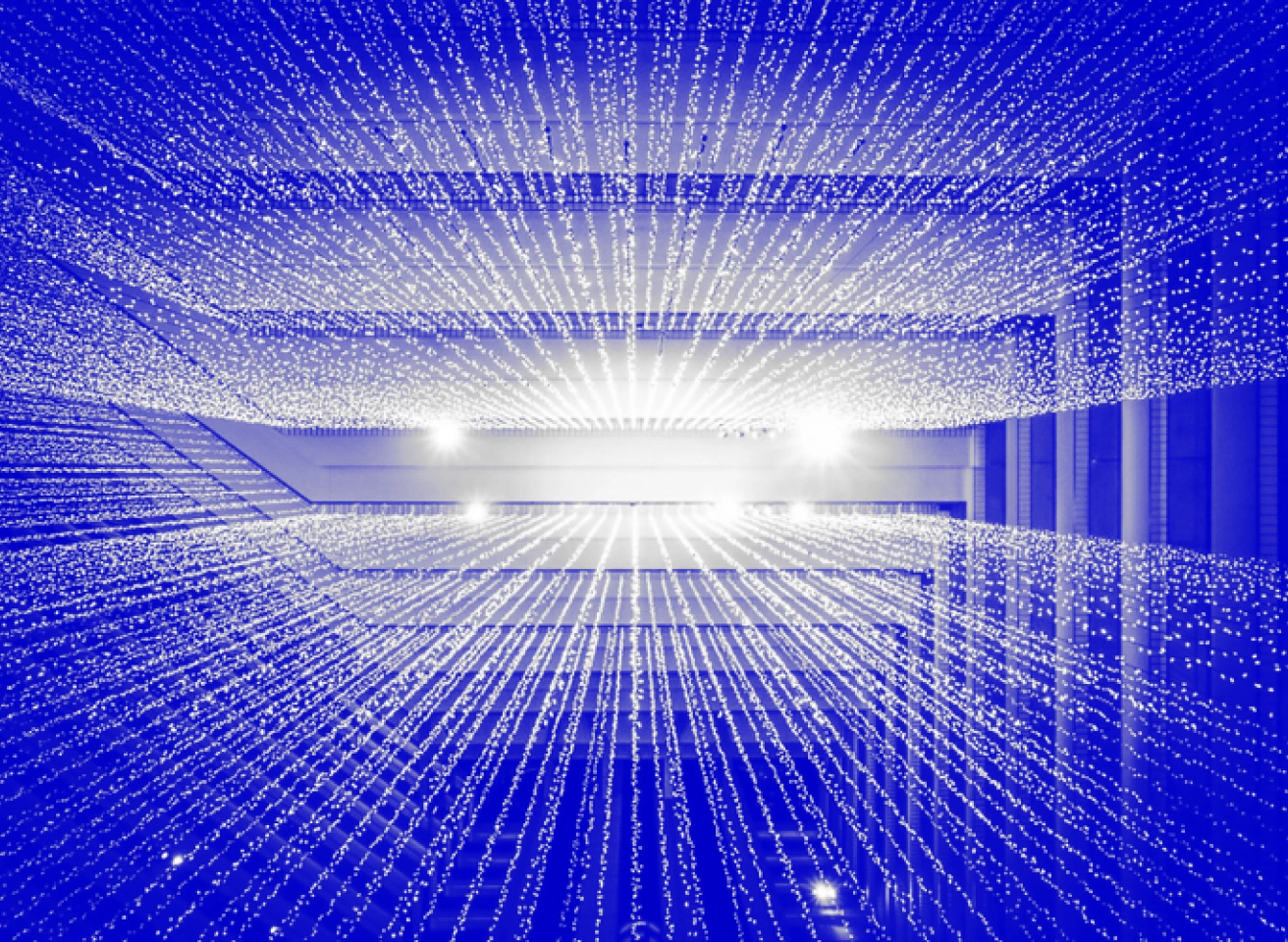
What Types of Tasks Can Be Solved with Data Science?
Data scientists work with different business needs to discover insights from existing data. There is no single technology that encompasses data science. Different tasks require different technologies, and, very often, several of them. In this article, we discuss the main tasks facing data scientists when solving problems for businesses.
Read article