Real Life Data Science Applications in Healthcare
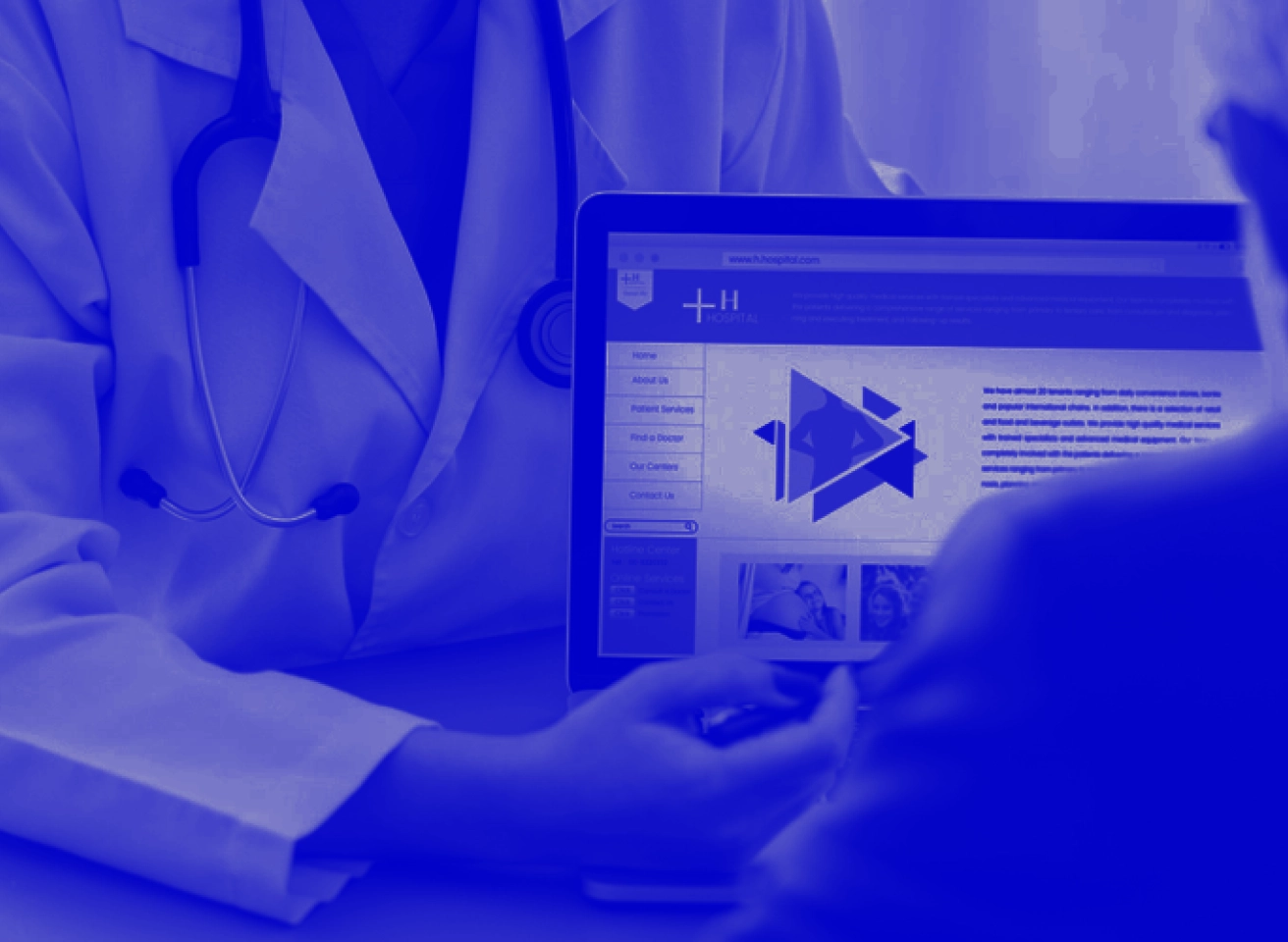
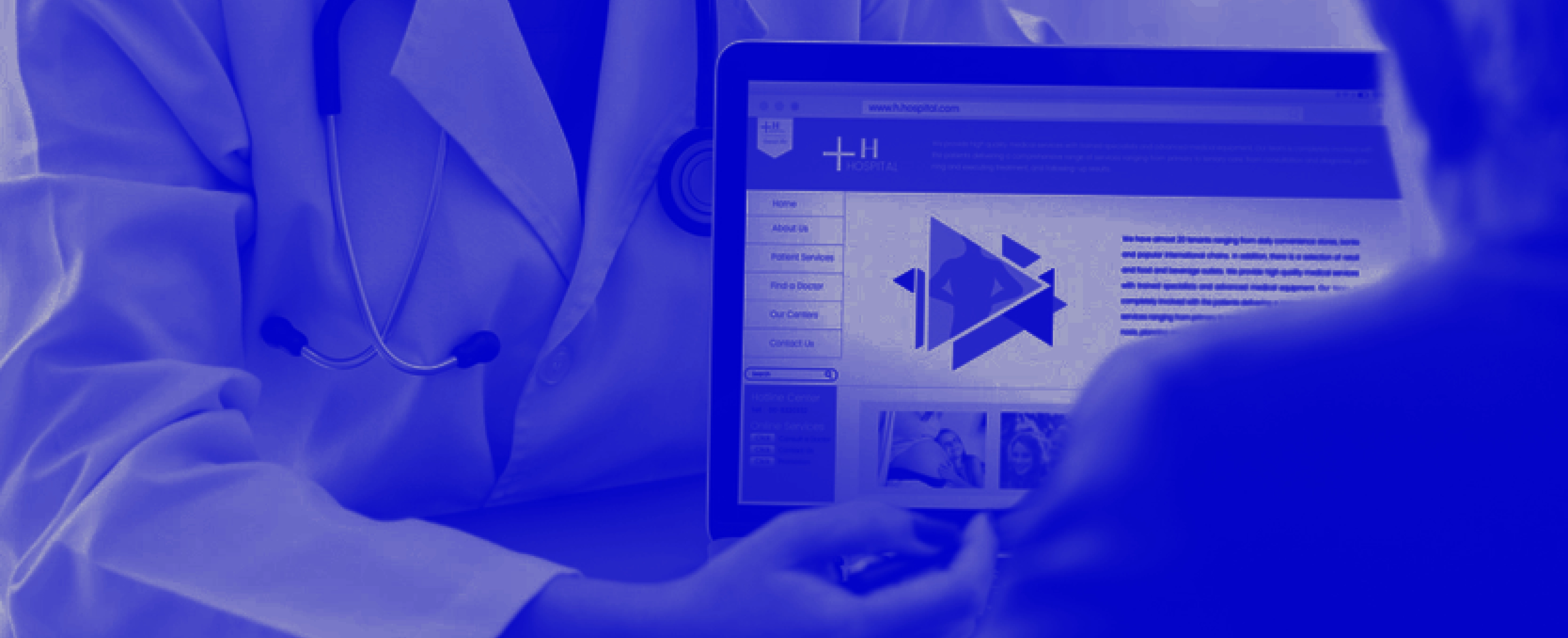
Due to healthcare's importance to humanity and the amount of money concentrated in the industry, its representatives were among the first to see the immense benefits to be gained from innovative data science solutions. For healthcare providers, it’s not just about lower costs and faster decisions. Data science also helps provide better services to patients and makes doctors' work easier. But that’s theory, and today we’re looking at specifics.
Health Information Systems
Health information systems collect patient data and compile it in a way that can be used to make quick and accurate healthcare decisions. In hospitals, Clinical Decision Support (CDS) software analyzes medical data on the spot and provides advice for practitioners. For example, if a patient’s blood pressure increases too much, the system will send a real-time alert to their doctor.
And since there’s plenty of money in the healthcare industry, numerous startups develop systems for individual usage outside hospitals. These products use patients’ data, in conjunction with data from CDS, in order to create better treatment plans for people with asthma, diabetes, and other diseases. Algorithms based on deep learning increase diagnostic accuracy by analyzing previous examples and then suggesting better treatment solutions.
Hospitals collect patients’ digital medical history for what is called an Electronic Health Record (EHR) (or Electronic Medical Record, EMR). An EHR includes demographics, medical history, treatments, and test results. It can send out reminders when a patient needs to get a new lab test or track if a patient follows their doctor’s orders.
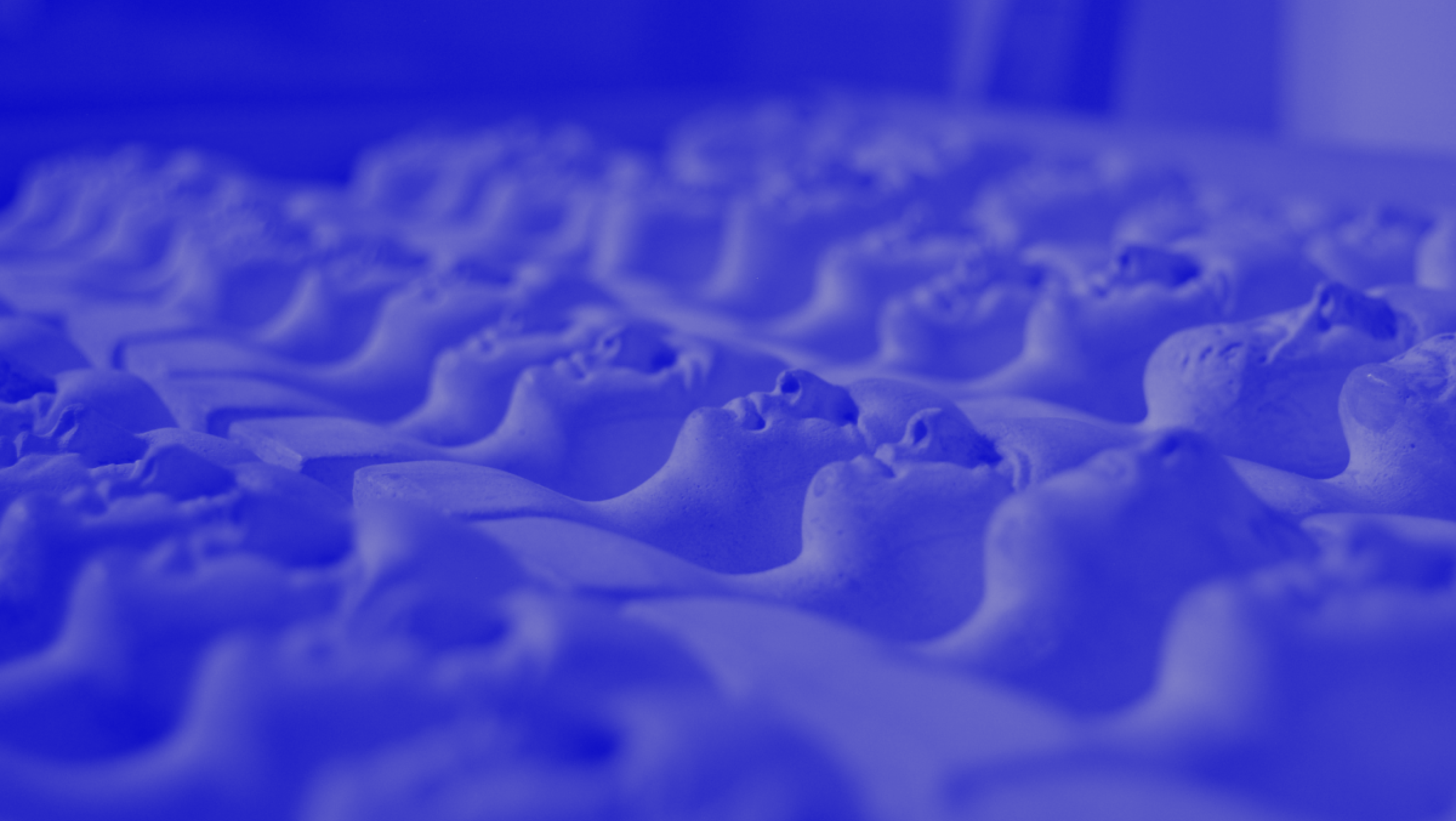
1 Real Life Data Science Applications in Healthcare
Integrating EHR with CDS presents great opportunities for improving patient diagnosis and reducing visits to hospital; this combination can also be be used for preventing diseases. For example, EMR was used to predict myopia progression in East and Southeast Asia, which affected an estimated 80%–90% of high school graduates. The algorithm was able to predict which children were at high risk of getting sick. This way, preventative measures could be taken and the epidemic could be stopped.
Drug Discovery
Producing new drugs costs billions of dollars and takes years of time (twelve years on average to get a drug officially submitted). Machine learning algorithms and AI already simplify this process by reducing the number of required lab experiments for metabolic-disease therapies, cancer treatment, immuno-oncology drugs, etc. Time reduction is especially important in the case of an acute epidemiological situation.
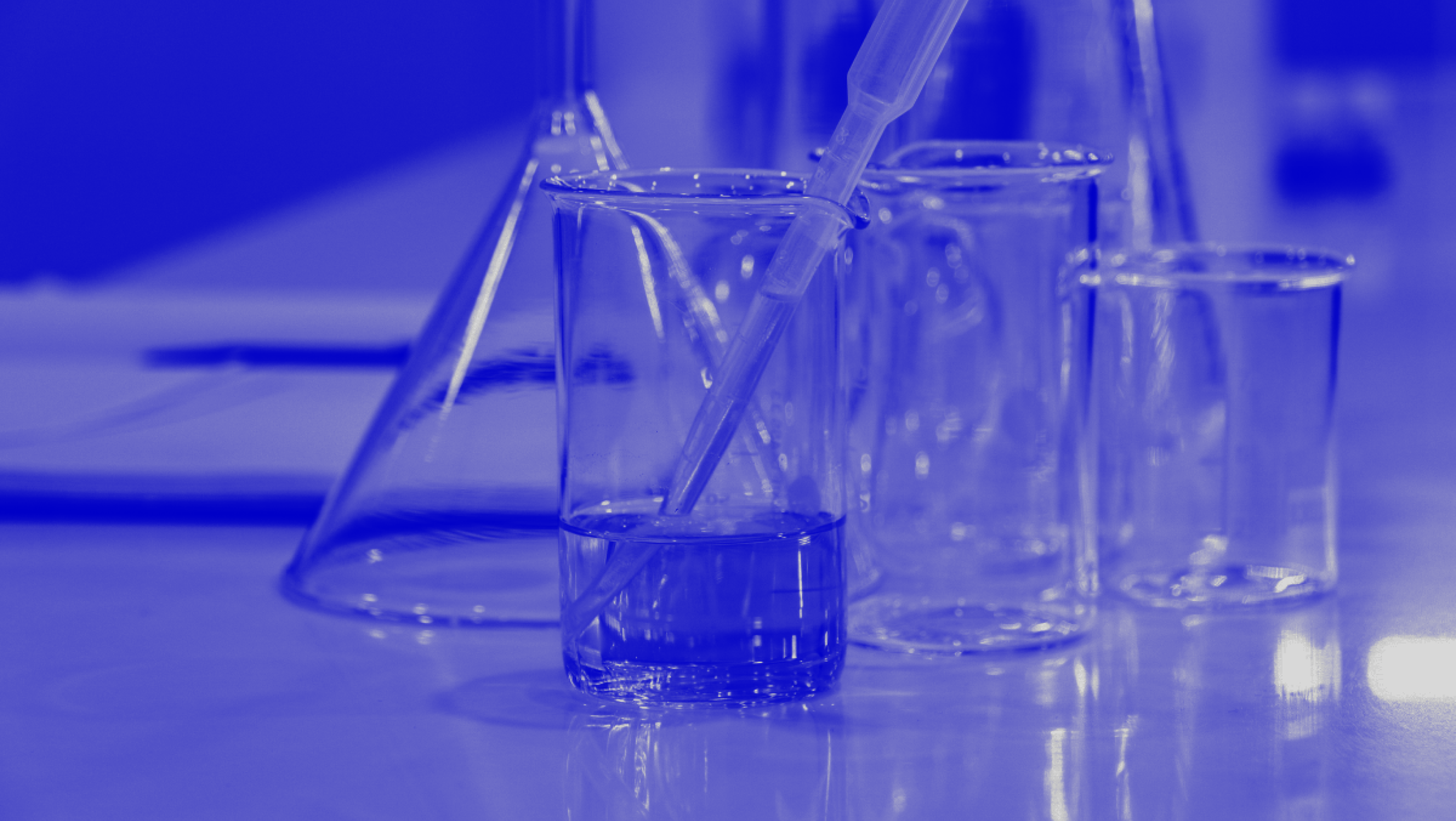
2 Real Life Data Science Applications in Healthcare
Medical Image Analysis
Medical equipment (X-ray CT, MRI/fMRI, etc.) produces complex and highly informative 2- and 3-dimensional images. Analyzing big volumes of raw visual data is extremely time-consuming. This is a big reason why data science has become one of the major tools of medical image analysis.
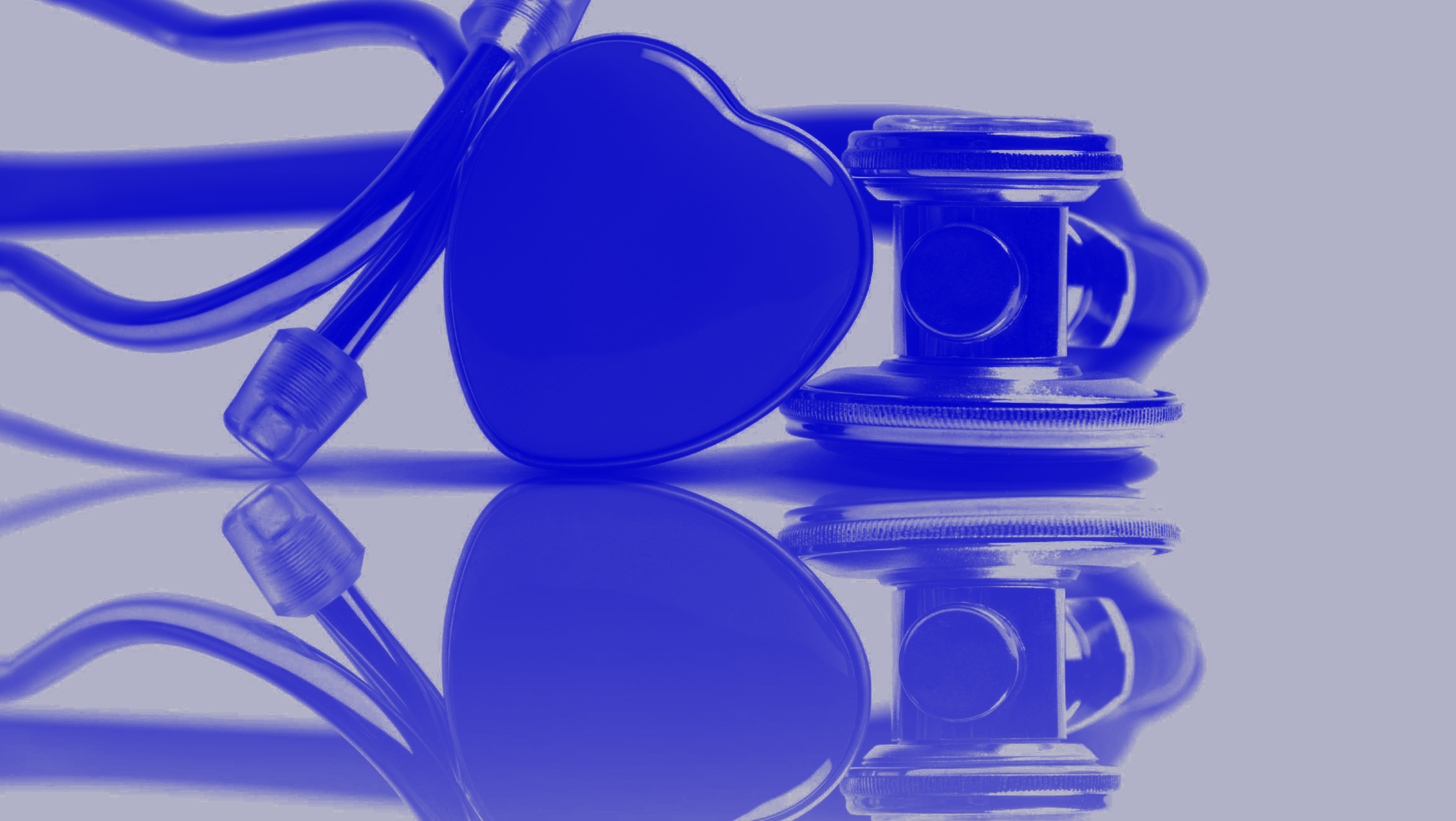
3 Real Life Data Science Applications in Healthcare
Medical image analysis
Machine learning methods, content based medical image indexing, and wavelet analysis for solid texture classification are used to detect tumors, artery stenosis, organ delineation, etc. It is important to note that systems based on machine learning detect diseases not only faster but also more accurately than humans. For example, an algorithm, called CheXNeXt, outperforms radiologists in disease detection using chest x-rays by 33% in accuracy.
Applications of Big Data in “-omics”
The organic and molecular fields, such as genomics, proteomics, metabolomics macrobiotics, and other types of “omics”, collect significant datasets. Data science techniques allow integration of different data types to realize the strategies of disease treatment and drug response. This type of approach enables advanced treatment personalization.
There are already more than a hundred applications that use genomics (MapReduce, Clue Go, GSEA, Pathaway-Express, etc.), and proteomics (MaxQuant, Mascot, SEQUEST, and many others) to help in preventing or curing diseases.
Microsoft HoloLens
This technology is an example of using AI in surgery. HoloLens combines elements of virtual reality and augmented reality to map the anatomy of a patient and overlay 3D images of blood vessels, bones, and organs on top of their limbs. This pre-operate procedure helps to “see” the unique anatomy of each patient and plan the operation accordingly.
Conclusions
The usage of healthcare data analytics in medical institutions is growing extremely fast. The main reasons for this are:
- High volumes of data which require real-time analysis.
- Handling complex data demands special analytical techniques.
- Data science provides efficient data collection technologies.
Data science techniques in the healthcare system are going to play a vital role in how healthcare is practiced in the future.
Interesting For You
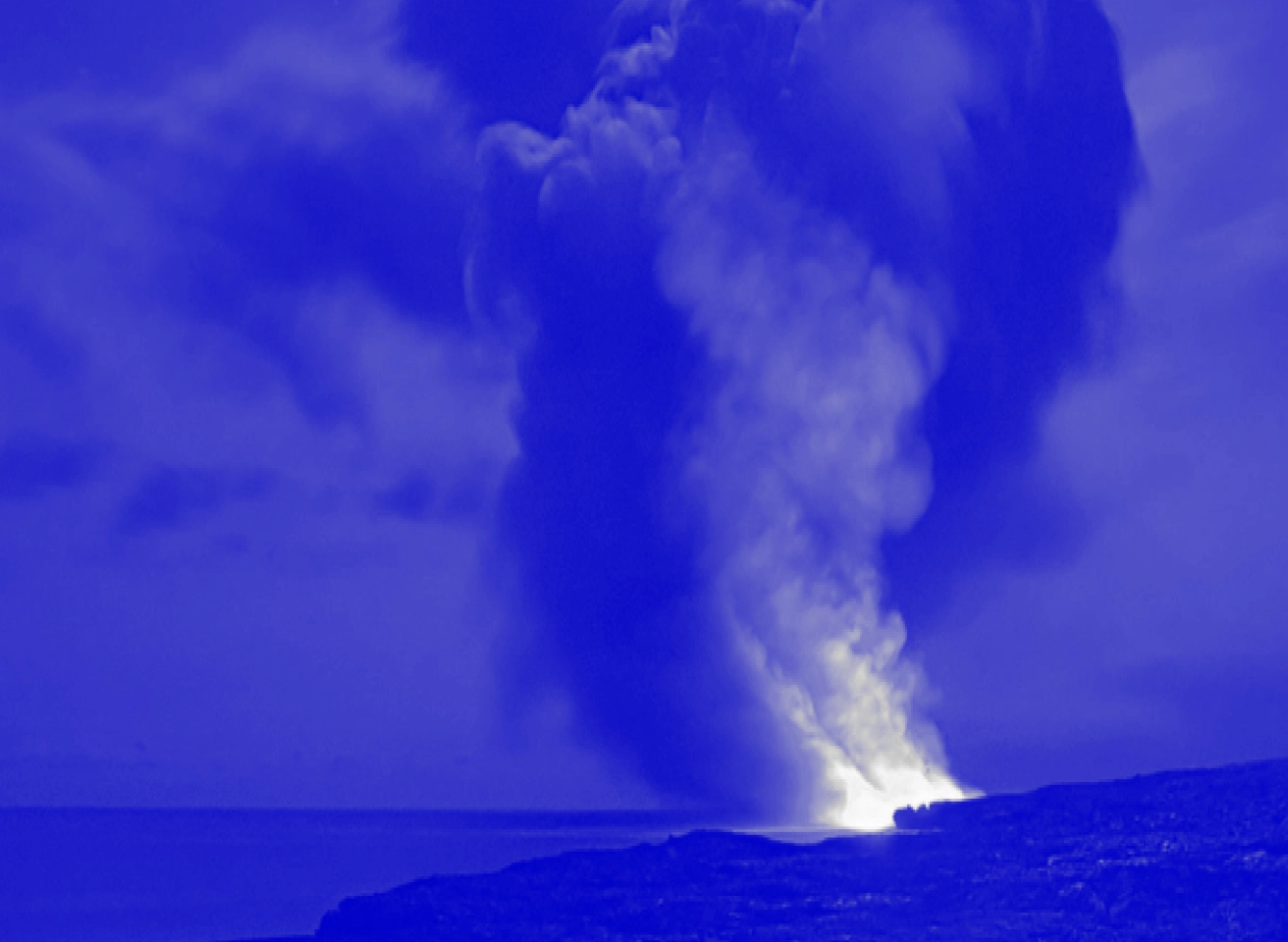
Data Science Usage in Natural Disasters Predictions
Millions of people are affected by natural disasters each year. Wildfires, floods, tornadoes, volcanic eruptions, are just the beginning of a long list of potential disasters. Some can last a few seconds, while others can last for weeks. However, their effects can be felt for decades or even longer, and impact the global economy, infrastructure, agriculture, and human health. The worst part is that the future impact of disasters will grow dramatically due to climate change. Some regions, which previously rarely suffered floods or wildfires, now regularly experience the effects of these natural disasters. Researchers have collected a large amount of data and developed models that predict disasters, but most of these models are far from perfect. For instance, the amount of data that is monitored by satellites and various ground sensors all over the world each minute is incredibly large, and therefore presents a major challenge for researchers. Having lots of information can be an asset, but data requires computational resources. As more data is collected, computational models become increasingly complex and slow. Furthermore, since just a few minutes’ notice in advance of a flood or wildfire can save people’s lives, predictive models must be able to work and do corrections in real time. Artificial Intelligence (AI) techniques and approaches, like data mining, machine learning, and deep learning, can assist in disaster prediction. It is possible for AI to find hidden dependencies in data, which can be a basis for better understanding the mechanism of disasters, and, as a result, making better predictions. Good predictions and warnings reduce economic losses and save lives. We can’t stop most disasters, like floods, hurricanes, volcano eruptions, but we can be prepared for them. In this article, we will illustrate how data science can help in predicting different natural disasters.
Read article
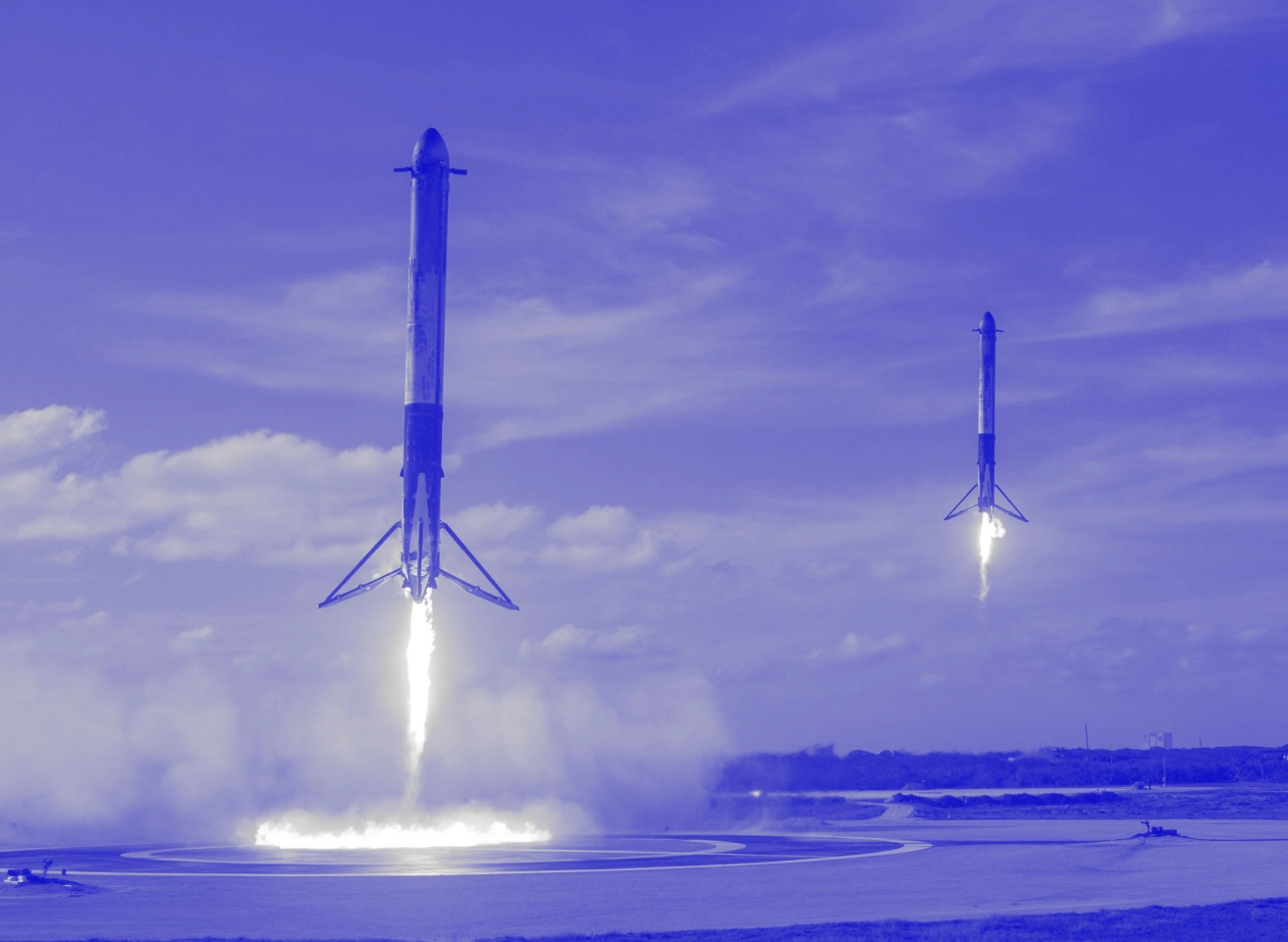
Predictive Analysis in Business
Decision-making in business is often based on assumptions about the future. Many companies aspire to develop and deploy an effective process for understanding trends and relationships in their activity in order to gain forward-looking insight to drive business decisions and actions. This is called predictive analytics. We can define predictive analytics as a process that uses data and a set of sophisticated analytic tools to develop models and estimations of an environment's behavior in the future. In predictive analysis, the first step is to collect data. Depending on your target, varied sources are using, such as web archives, transaction data, CRM data, customer service data, digital marketing and advertising data, demographic data, machine-generated data (for example, telemetric data or data from sensors), and geographical data, among other options. It is important to have accurate and up to date information. Most of the time, you will have information from multiple sources and, quite often, it will be in a raw state. Some of it will be structured in tables, while the rest will be semi-structured or even unstructured, like social media comments. The next important step is to clean and organize the data - this is called data preprocessing. Preprocessing usually takes up 80% of the time and effort involved in all analysis. After this stage, we produce a model using already existing tools for predictive analytics. It is important to note that we use collected data to validate the model. Such an approach is based on the main assumption of predictive analytics, which claims that patterns in the future will be similar to the ones in the past. You must ensure that your model makes business sense and deploy the analytics results into your production system, software programs or devices, web apps, and so on. The model can only be valid for a certain time period, since reality is not static and an environment can change significantly. For example, the preferences of customers may change so fast that previous expectations become outdated. So, it is important to monitor a model periodically. There are plenty of applications for business based on predictive analytics. To conclude this article, we will briefly consider some of them.
Read article
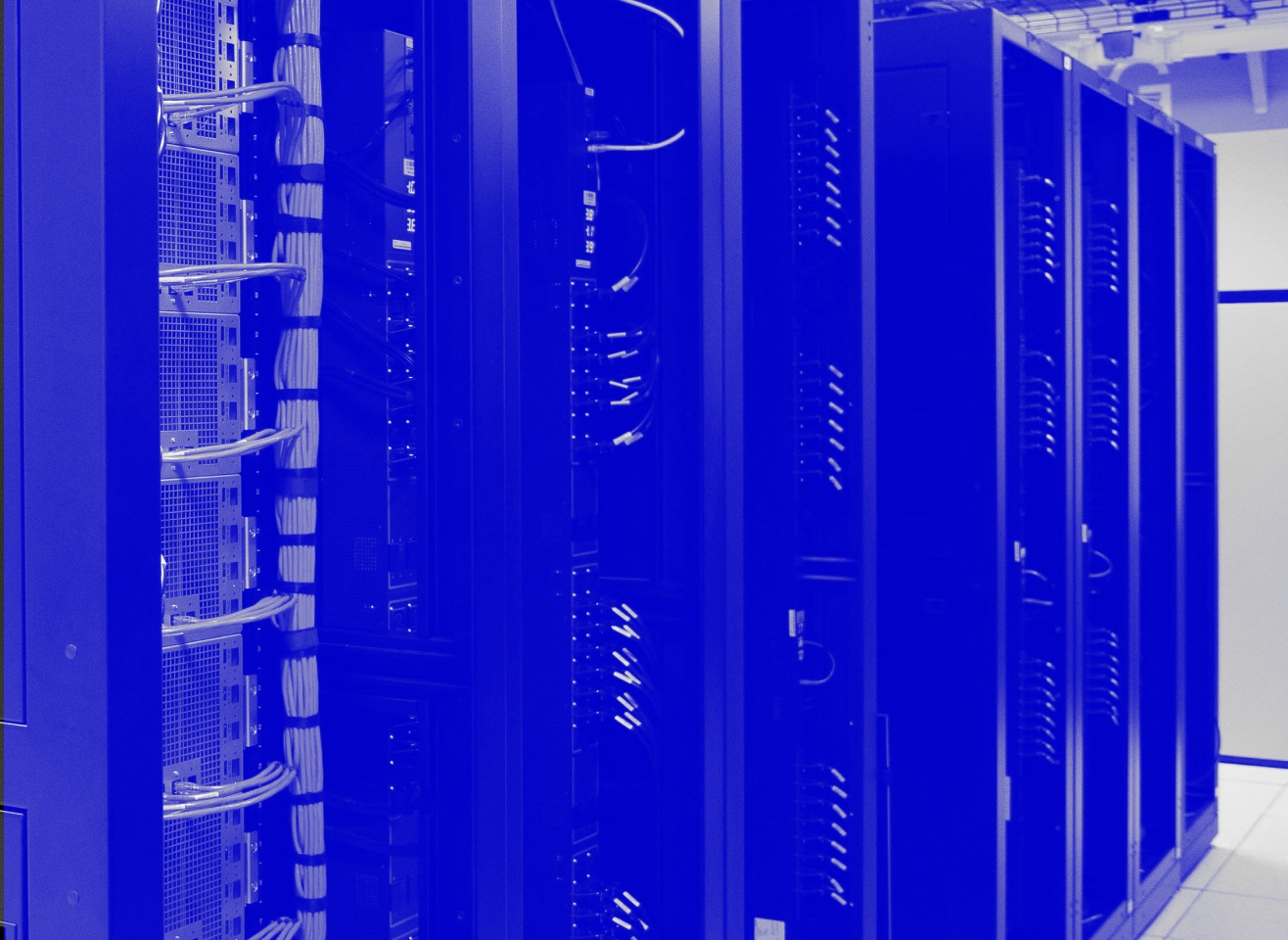
How Can Data Science Help My Organization?
Nowadays, there is a tendency to hire data scientists or even form data science groups in companies. This does not only apply to specific activity sectors or large organizations. Small and midsize businesses are more frequently involving data scientists, in order to get actionable insights from collected information. So, how does data help to run and grow everyday businesses? There are several areas where collected data and the insights drawn from that data can have a significant impact on business.
Read article