Predictive Analysis in Business
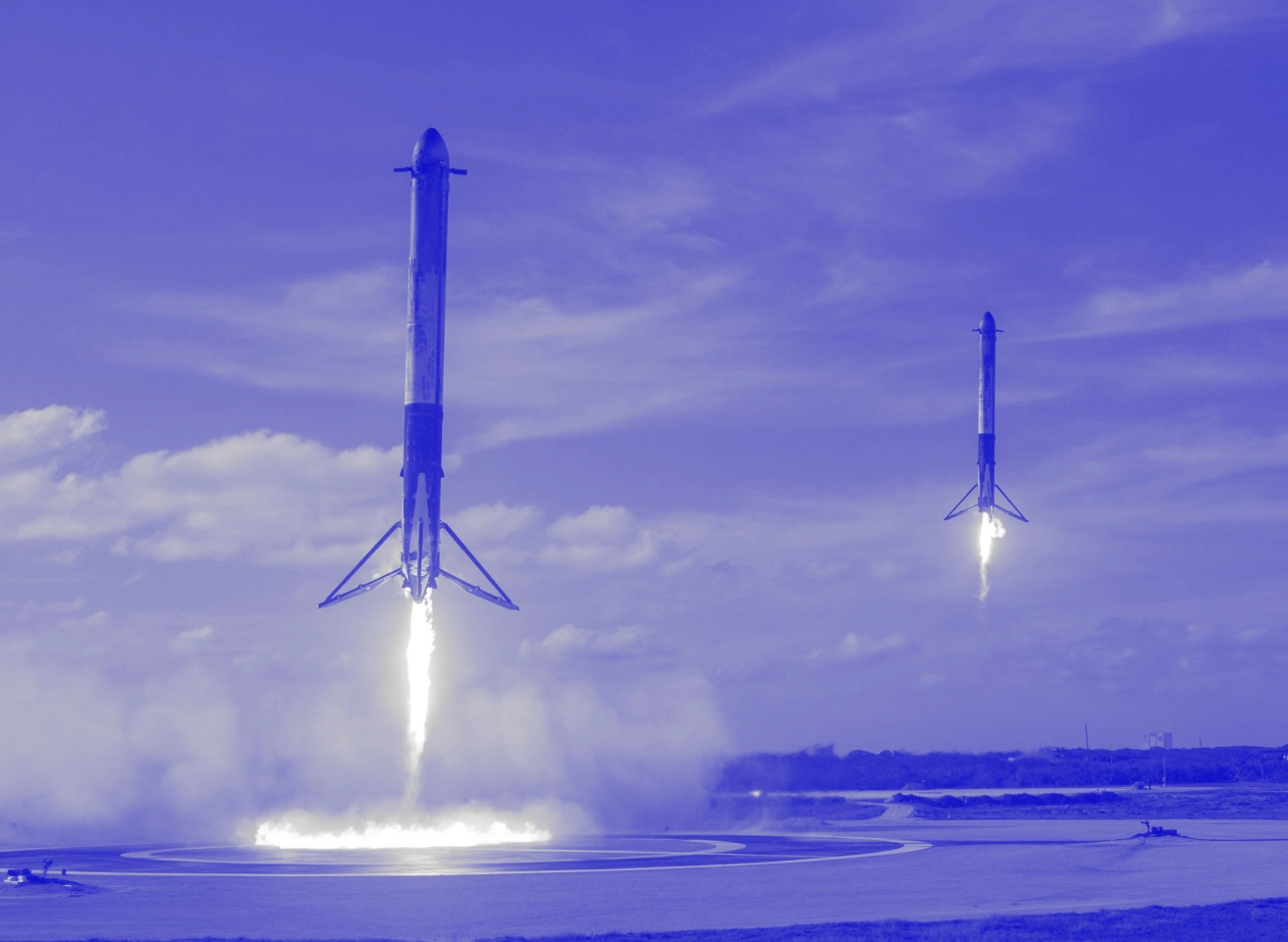
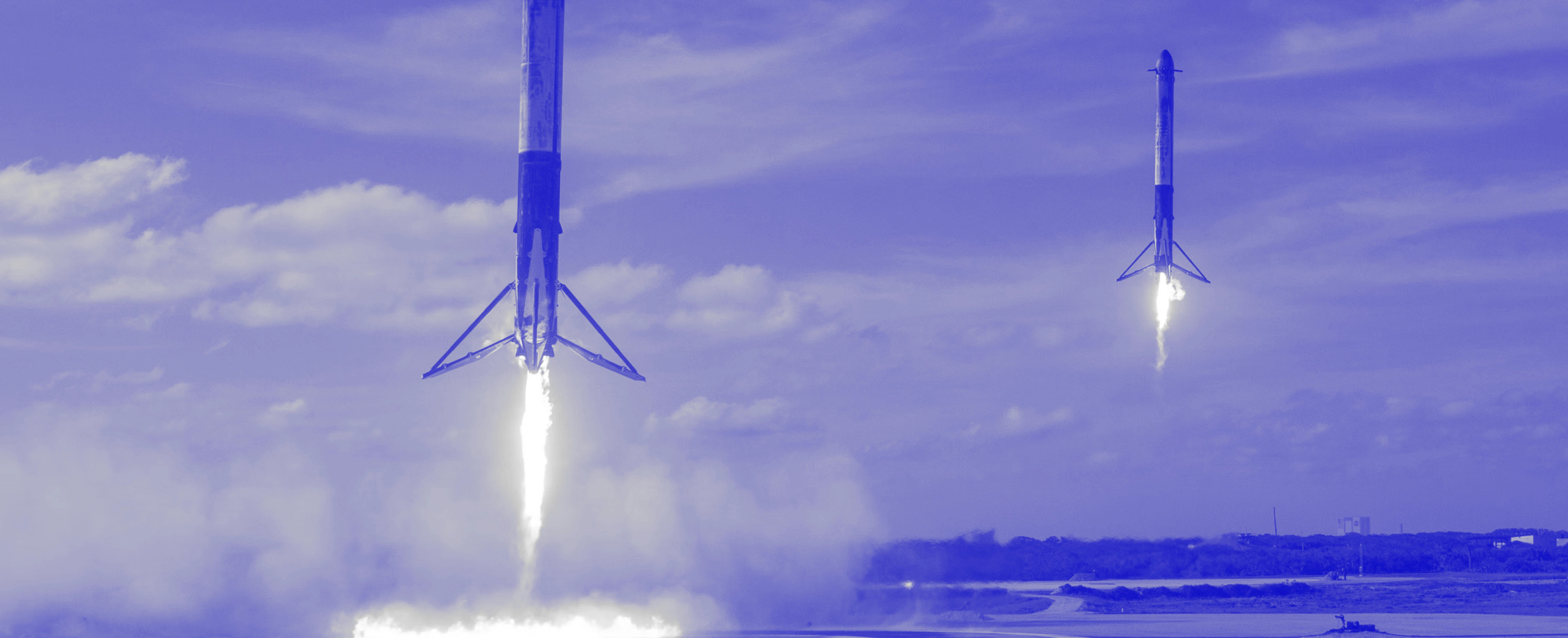
Decision-making in business is often based on assumptions about the future. Many companies aspire to develop and deploy an effective process for understanding trends and relationships in their activity in order to gain forward-looking insight to drive business decisions and actions. This is called predictive analytics. We can define predictive analytics as a process that uses data and a set of sophisticated analytic tools to develop models and estimations of an environment's behavior in the future. In predictive analysis, the first step is to collect data. Depending on your target, varied sources are using, such as web archives, transaction data, CRM data, customer service data, digital marketing and advertising data, demographic data, machine-generated data (for example, telemetric data or data from sensors), and geographical data, among other options. It is important to have accurate and up to date information. Most of the time, you will have information from multiple sources and, quite often, it will be in a raw state. Some of it will be structured in tables, while the rest will be semi-structured or even unstructured, like social media comments. The next important step is to clean and organize the data - this is called data preprocessing. Preprocessing usually takes up 80% of the time and effort involved in all analysis. After this stage, we produce a model using already existing tools for predictive analytics. It is important to note that we use collected data to validate the model. Such an approach is based on the main assumption of predictive analytics, which claims that patterns in the future will be similar to the ones in the past. You must ensure that your model makes business sense and deploy the analytics results into your production system, software programs or devices, web apps, and so on. The model can only be valid for a certain time period, since reality is not static and an environment can change significantly. For example, the preferences of customers may change so fast that previous expectations become outdated. So, it is important to monitor a model periodically. There are plenty of applications for business based on predictive analytics. To conclude this article, we will briefly consider some of them.
Fraud and Risk Detection
Historically, this is one of the first applications of data science in business. Available data is used to identify non-obvious fraud or risk patterns, then business operations are monitored to detect such patterns. For example, banking companies employ big data methodologies for predictive fraud propensity models and then use them to create alerts that help ensure timely responses when unusual data is recognized. Airline companies use predictive analytics to predict flight delays.

1 Predictive Analysis in Business
Management Improving
Insights on client behavior can be used to implement better management. For example, you can define how many people you need to put on staff at any time period to improve customer service. Some public hospitals in Paris use data to predict the daily and hourly number of patients at each hospital.uation.
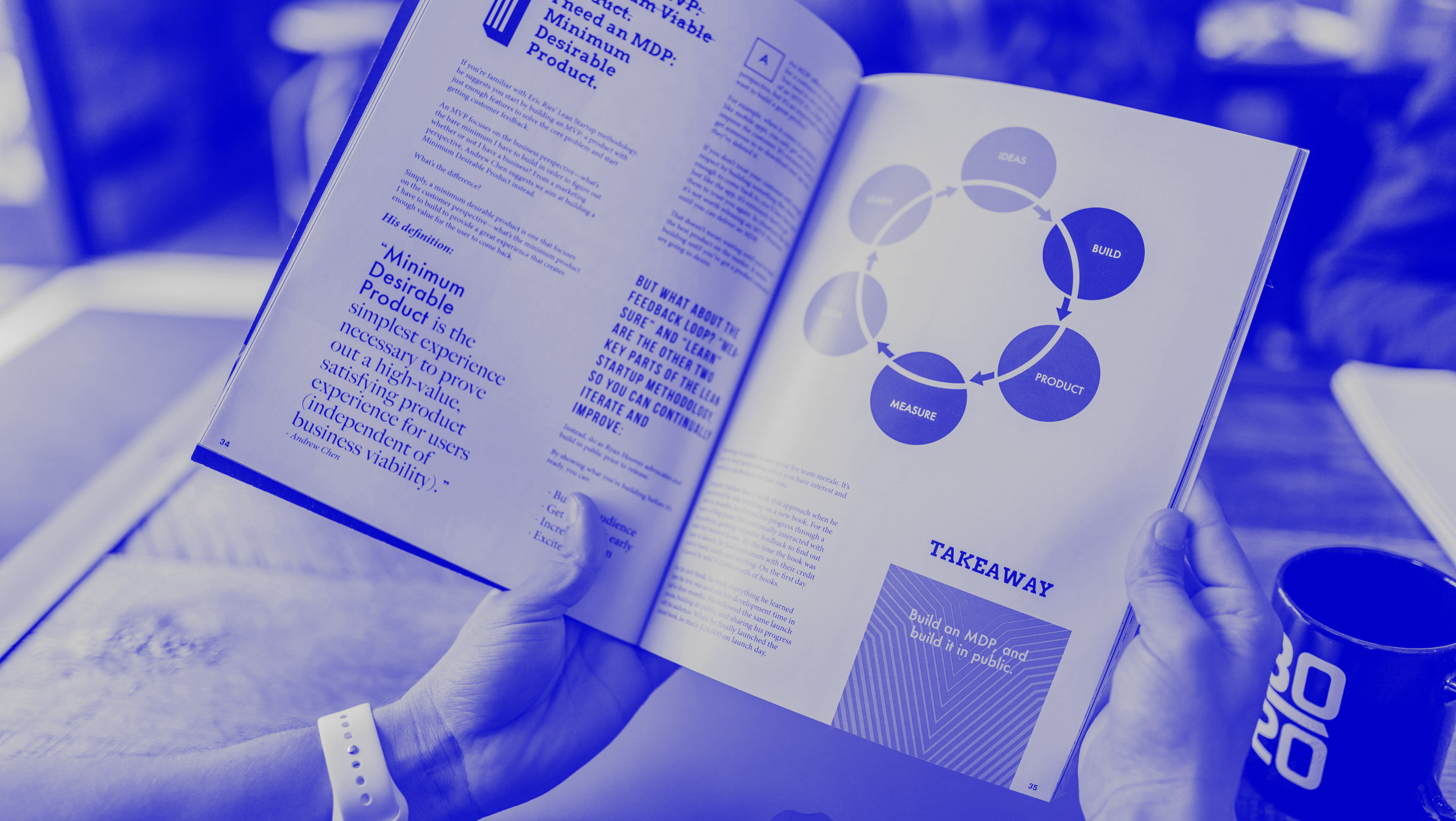
2 Predictive Analysis in Business
Times Series Forecasting
Time series forecasting is a technique that is used to predict future values based on previously observed values. It is widely used in finance, in supply chain management, production and inventory planning. For example, companies can accurately predict product demand by looking at different types of factors: prior history, seasonality, and market-moving events. Such analysis is used to realistically plan for sales.
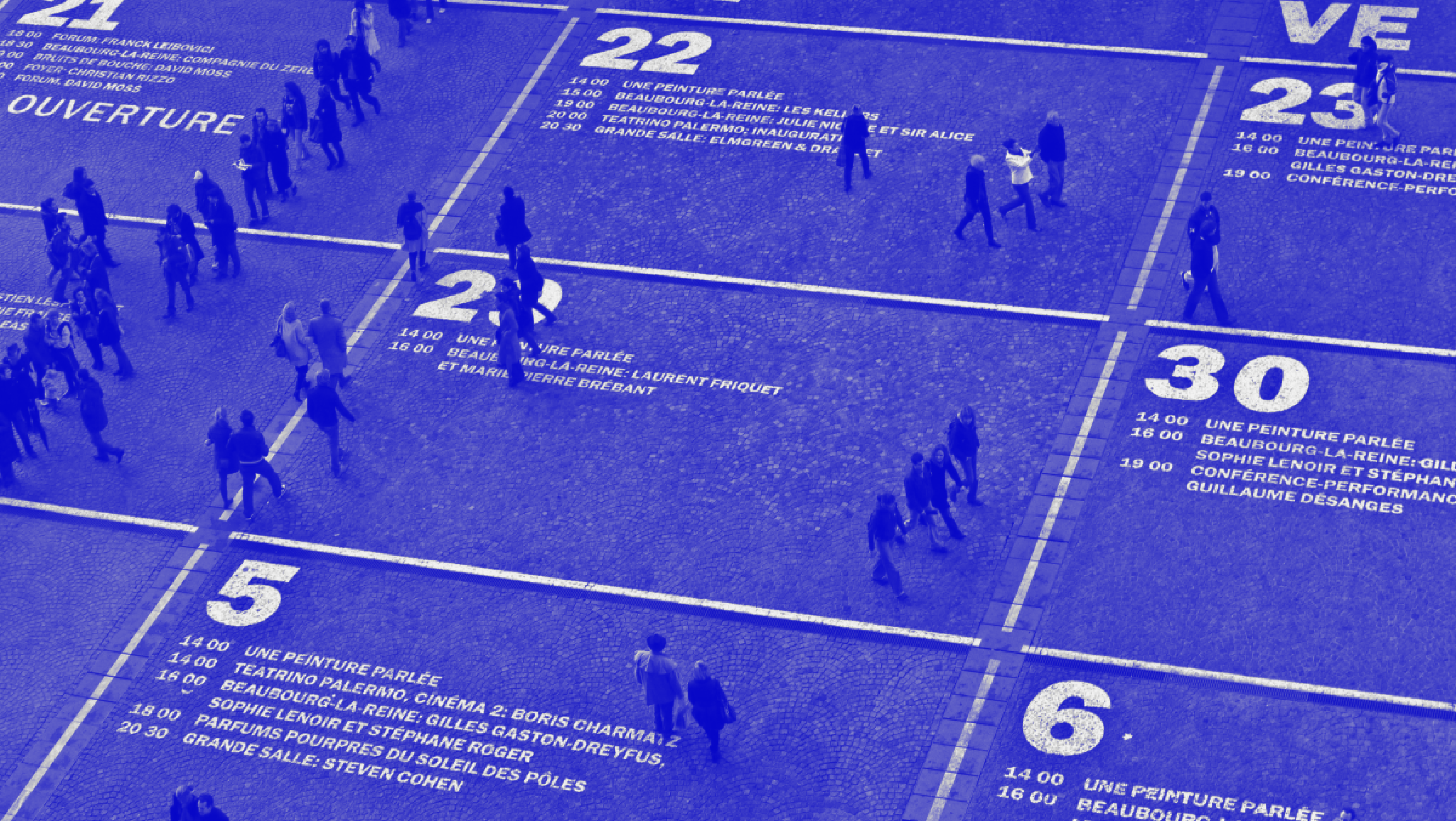
3 Predictive Analysis in Business
Refining Marketing Strategy
Customer data analysis helps companies to understand how consumers are engaging with and responding to their marketing campaigns. Thus, companies can find new target markets that they can capitalize on. In this regard, the use of predictive analytics provides valuable insights on how to sell a product to those who need it at a reasonable cost, at the right time and using the right channel.

4 Predictive Analysis in Business
Interesting For You
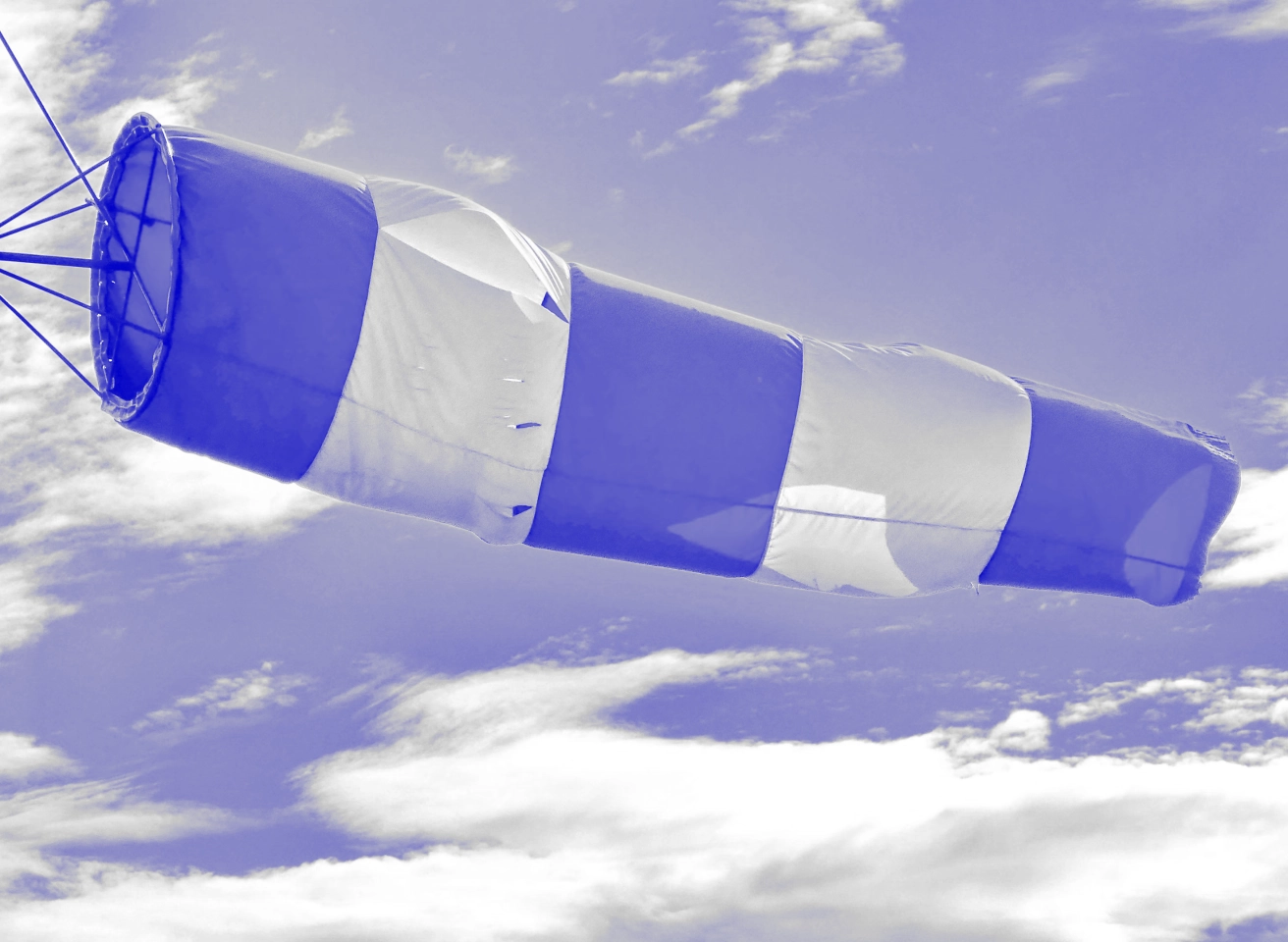
Predictive Analytics Workflow
Many companies use predictive models in their activity to provide better customer service, sell more products and services to customers, manage risk from fraudulent activity, and better plan the use of their human resources (to list a few important examples). How does predictive analysis offer all of these benefits? In this article we will consider the process of predictive analytics, and its related advantages.
Read article
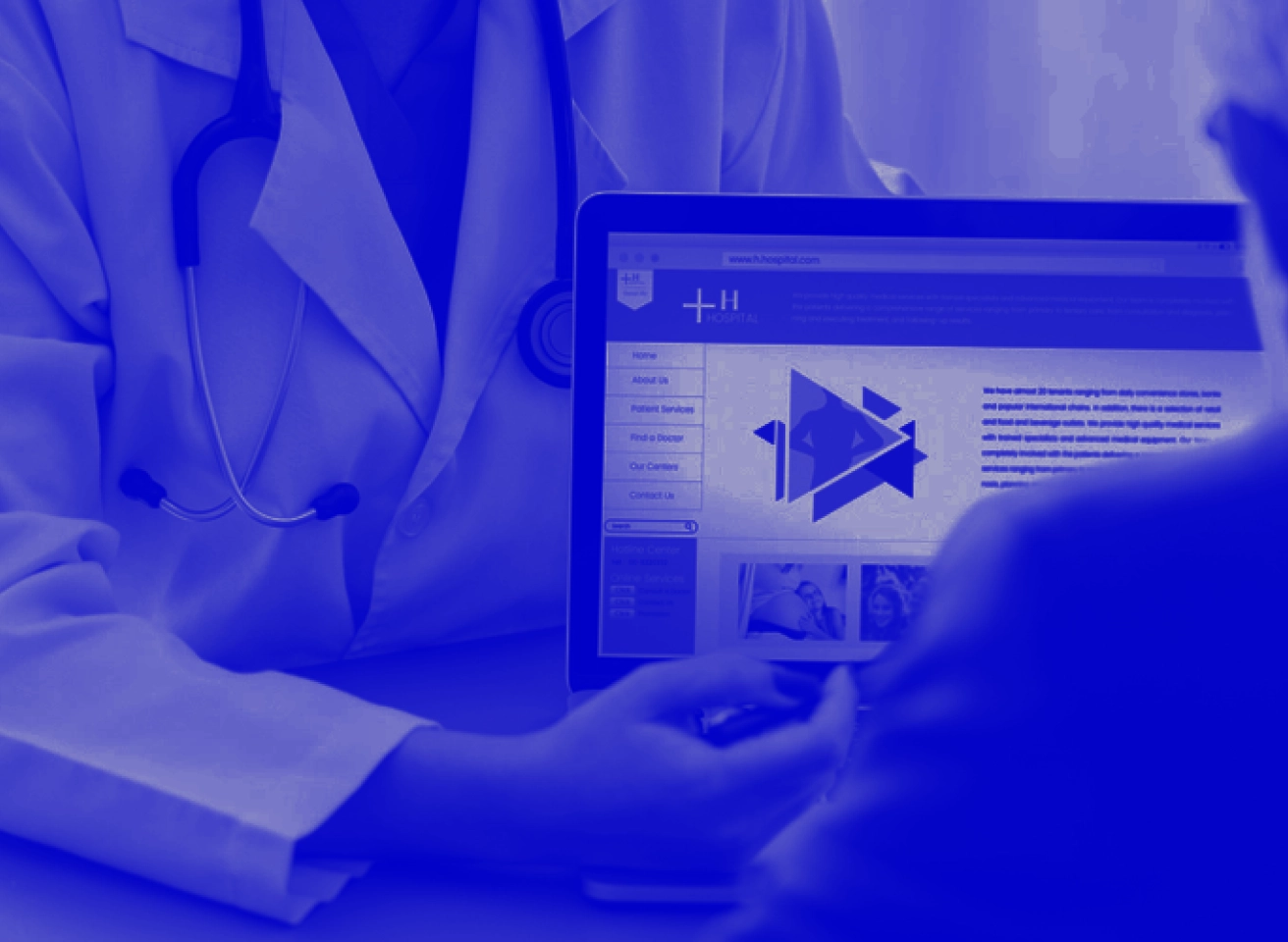
Real Life Data Science Applications in Healthcare
Due to healthcare's importance to humanity and the amount of money concentrated in the industry, its representatives were among the first to see the immense benefits to be gained from innovative data science solutions. For healthcare providers, it’s not just about lower costs and faster decisions. Data science also helps provide better services to patients and makes doctors' work easier. But that’s theory, and today we’re looking at specifics.
Read article
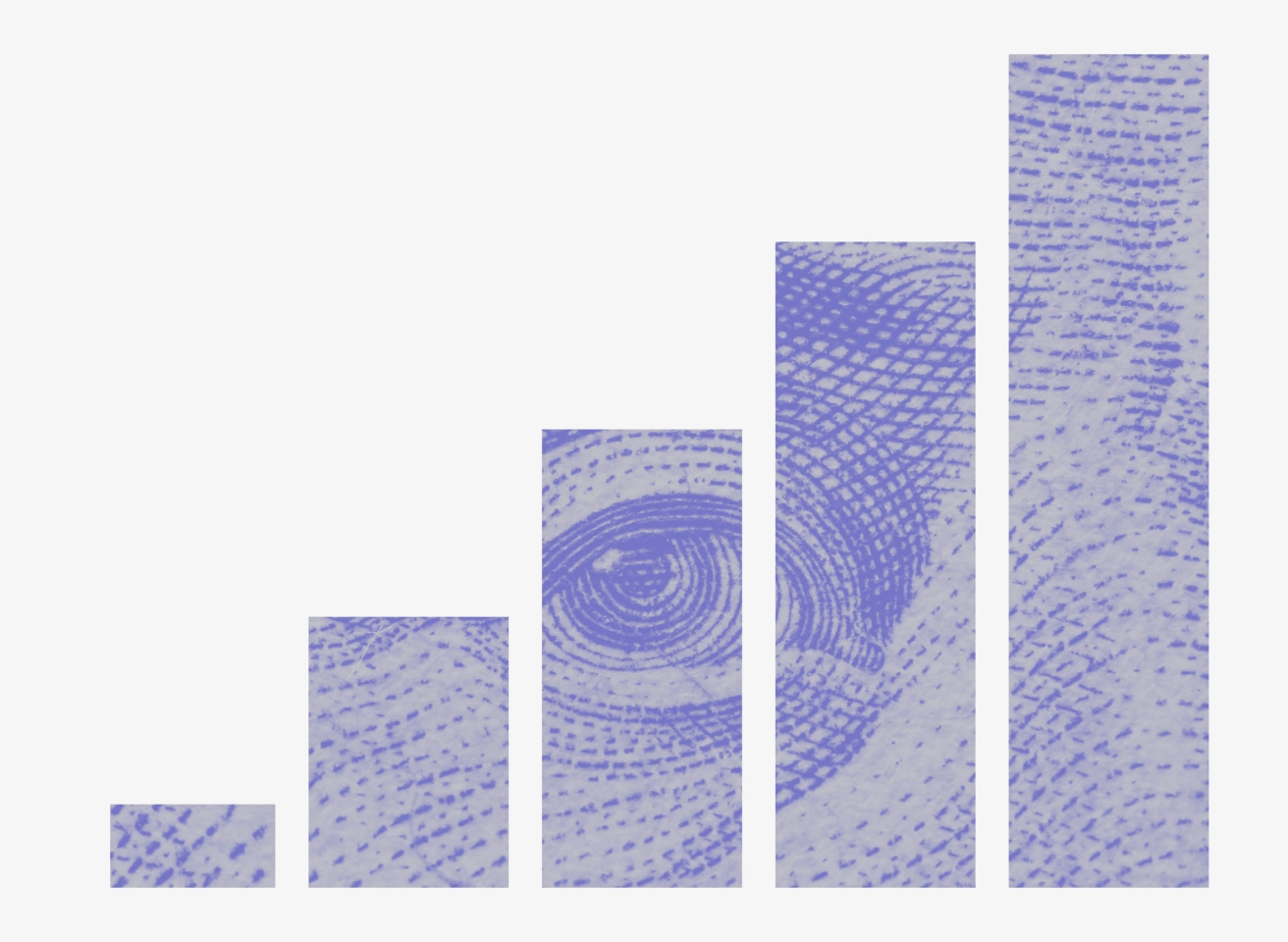
Data Science in E-Commerce
More than 20 years ago, e-commerce was just a novel concept, until Amazon sold their very first book in 1995. Nowadays, the e-commerce market is a significant part of the world’s economy. The revenue and retail worldwide expectations of e-commerce in 2019 were $2.03 trillion and $3.5 trillion respectively. This market is developed and diverse both geographically and in terms of business models. In 2018, the two biggest e-commerce markets were China and the United States, with revenues of $636.1 billion and $504.6 billion respectively. Currently, the Asia-Pacific region shows a better growth tendency for e-commerce retail in relation to the rest of the world. Companies use various types of e-commerce in their business models: Business-to-Business (B2B), Business-to-Consumer (B2C), Consumer-to-Consumer (C2C), Consumer-to-Business (C2B), Business-to-Government (B2G), and others. This diversity has emerged because e-commerce platforms provide ready-made connections between buyers and sellers. This is also the reason that B2B’s global online sales dominate B2C: $10.6 trillion to $2.8 trillion. Rapid development of e-commerce generates high competition. Therefore, it’s important to follow major trends in order to drive business sales and create a more personalized customer experience. While using big data analytics may seem like a current trend, for many companies, data science techniques have already been customary tools of doing business for some time. There are several reasons for the efficiency of big data analytics: · Large datasets make it easier to apply data analytics; · The high computational power of modern machines even allows data-driven decisions to be made in real time; · Methods in the field of data science have been well-developed. This article will illustrate the impact of using data science in e-commerce and the importance of data collection, starting from the initial stage of your business.
Read article