Data Science in E-Commerce
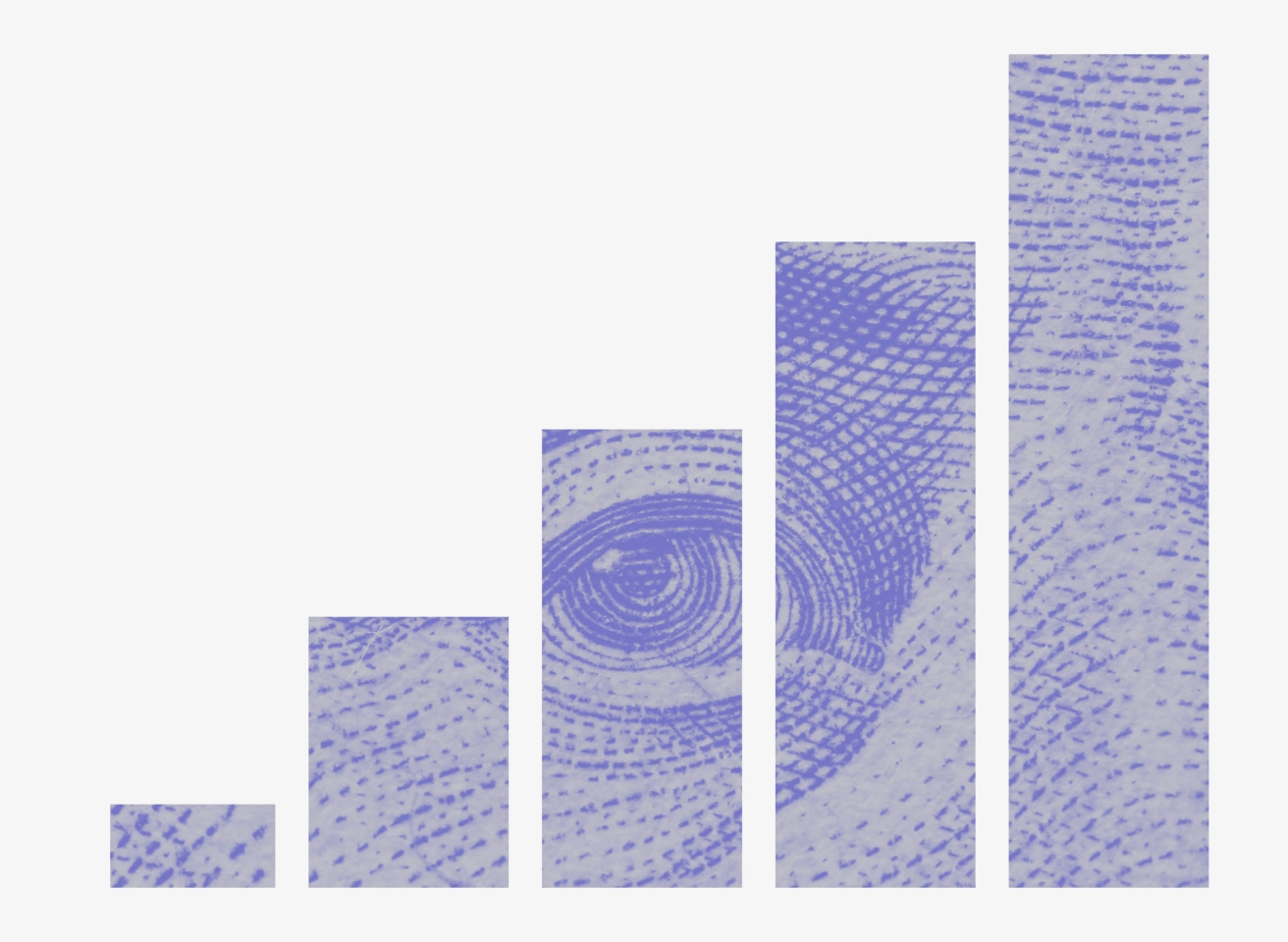
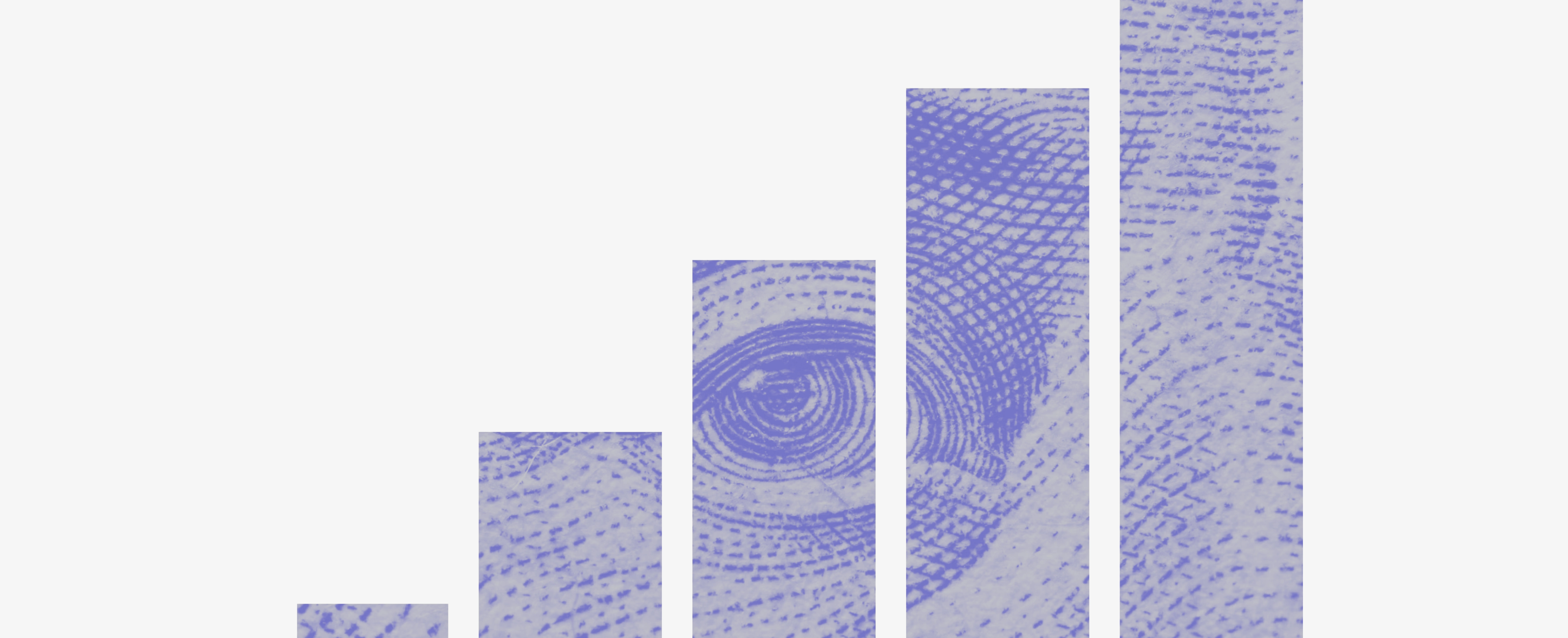
More than 20 years ago, e-commerce was just a novel concept, until Amazon sold their very first book in 1995. Nowadays, the e-commerce market is a significant part of the world’s economy. The revenue and retail worldwide expectations of e-commerce in 2019 were $2.03 trillion and $3.5 trillion respectively. This market is developed and diverse both geographically and in terms of business models. In 2018, the two biggest e-commerce markets were China and the United States, with revenues of $636.1 billion and $504.6 billion respectively. Currently, the Asia-Pacific region shows a better growth tendency for e-commerce retail in relation to the rest of the world. Companies use various types of e-commerce in their business models: Business-to-Business (B2B), Business-to-Consumer (B2C), Consumer-to-Consumer (C2C), Consumer-to-Business (C2B), Business-to-Government (B2G), and others. This diversity has emerged because e-commerce platforms provide ready-made connections between buyers and sellers. This is also the reason that B2B’s global online sales dominate B2C: $10.6 trillion to $2.8 trillion. Rapid development of e-commerce generates high competition. Therefore, it’s important to follow major trends in order to drive business sales and create a more personalized customer experience. While using big data analytics may seem like a current trend, for many companies, data science techniques have already been customary tools of doing business for some time. There are several reasons for the efficiency of big data analytics: Large datasets make it easier to apply data analytics; The high computational power of modern machines even allows data-driven decisions to be made in real time; Methods in the field of data science have been well-developed. This article will illustrate the impact of using data science in e-commerce and the importance of data collection, starting from the initial stage of your business.
Market Segmentation Analysis
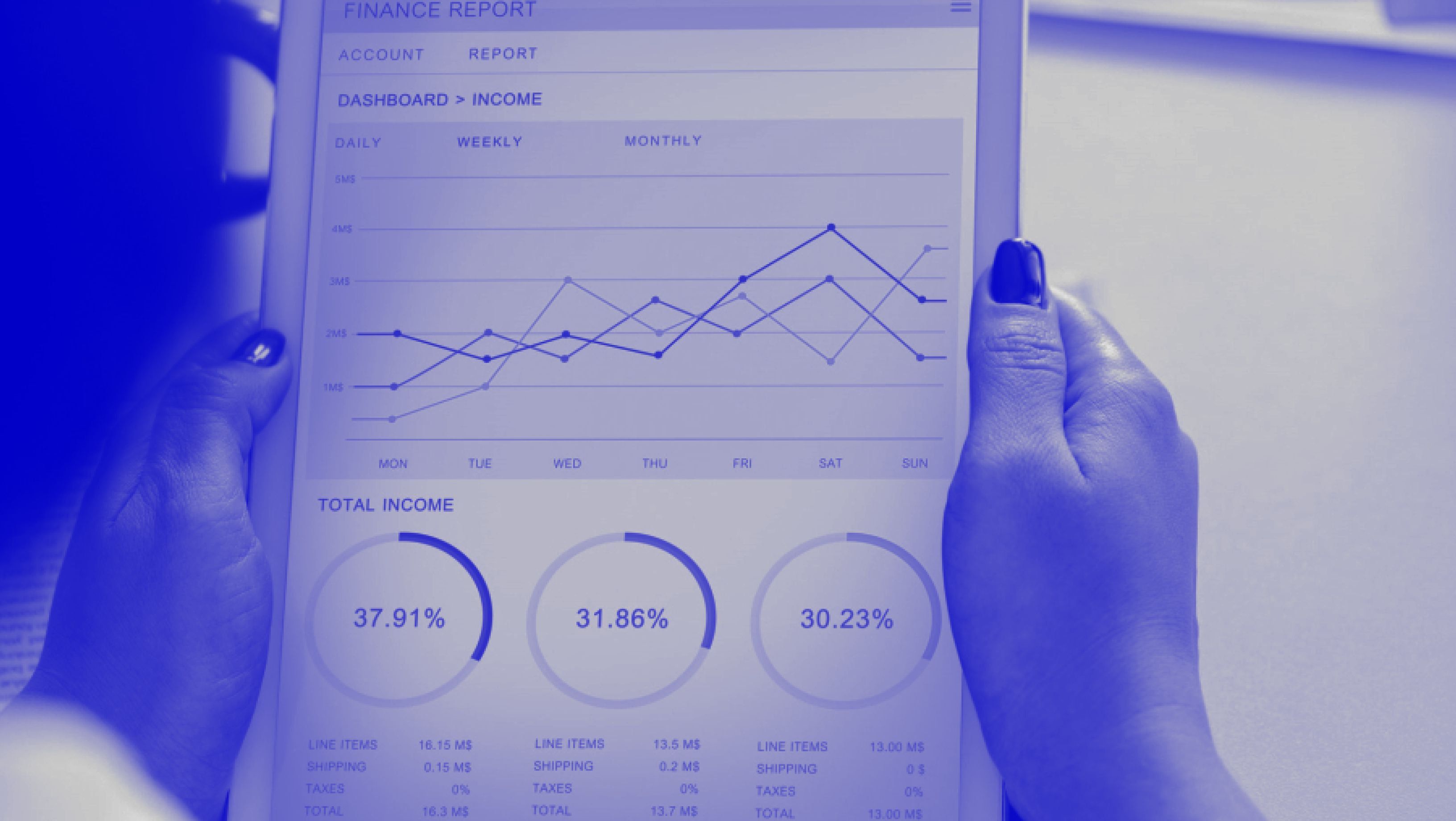
1 Data Science in E-Commerce
Strategic marketing planning is central to the development of any business. Market segmentation is one of the key building blocks of strategic marketing. In marketing segmentation, customers are subdivided into groups based on their attributes (age, gender, etc.). The aim of segmentation analysis is not only to identify different market segments, but also to develop a detailed profile, description and evaluation of the nature of each segment selected for targeting. Such an analysis can be done using data-driven analytics. The benefits of segmentation for a company are obvious:
· They can find segments which are better suited to their strengths, especially in comparison to competitors.
· They can identify what works for a particular segment and what doesn’t. Then, with the important knowledge of what is not working optimally, they can improve their product or service.
· Results allow them to better match their products or services to each segment’s needs.
The advantage of analysis based on data science methods is that this option makes it possible to analyze a variety of data. Earlier, segmentation was based on geographical data (country of origin, address), demography (age, gender, education). Now, we add to our analysis psychographic criteria (beliefs, interests, aspirations, etc.) and behavioral data (based on customers’ activity: amount spent, frequency of purchase, etc.). This makes analysis more complex but more detailed and precise. For example, instead of just “young females” or “high-educated French males” segments, data-driven segmentation finds “fashionista young females, who enjoy fine dining” or “auto addicted male, who play golf, enjoy wine-tastings” as segments. With the greater depth offered by this analysis, a company can better understand their customers and recognize more segments (up to 10-20). This is called micro-segmentation. Micro-segmentation is the basic key to understanding the motivation drivers behind customers’ actions (for example, why a customer may abandon their basket). Micro-segmentation-based management includes different dimensions, such as visit time and device preferences, which then allow companies to make predictions about customer behavior.
Recommendation System
One of the hottest trends in e-commerce is having a recommendation system. A recommendation engine filters information and predicts customers’ preferences in order to come up with relevant products. The filtering of information is personal to each customer and based on their past searches, as well as other customers’ searches and purchase data. Of course, processing large amounts of data coming from hundreds (or even millions) of users requires modern artificial intelligence techniques.
There are two categories of recommendation algorithms: collaborative filtering and content-based filtering. The first one uses data collected from a user’s activities on the website in order to find similarity with other users’ activities. For example, if a customer is looking for a new phone, the recommendation system will also suggest the purchase of a phone cover, because other users often buy a phone and phone cover together.
Content-based filtering finds recommendations based on comparison between the content of the product and a user profile. For example, a customer likes jazz music. So, the recommendation system on a music store website recommends the hottest jazz album to the user. There is also the option of combining the two filtering types mentioned above, resulting in what is called hybrid recommendation filtering. Usually, we can take full advantage of the hybrid technique when there is more information about users available. In hybrid filtering, predictions of one technique are used as input for another technique.
Websites with a recommendation engine have a powerful advantage compared to those without one: namely, they can recommend to their customers what customers didn’t know they wanted. Amazon says its recommendation system generates 20-35% of the company's revenue.
Customer Lifetime Value (LTV) Modeling
In any business, it is very important to focus marketing efforts on the right channels. Machine learning methods allow companies to predict customer lifetime value (LTV). LTV refers to the value that each customer will bring to the company’s revenue. The model uses information about a customer’s needs, expenses, and recent purchases, in order to (a) make an estimation of how long a person has remained a customer and (b) predict their future purchases. This analysis helps e-commerce businesses in several ways:
· Identification and care for high-value customers.
· Estimation of future sales and defining growth.
· Adjusting campaign and advertisement strategies.
Churn Model
The churn model is a commonly-used model that helps to achieve customer retention. Getting engagement from new customers is good, but it is more expensive than maintaining relationships with existing customers. Customer churn prediction discovers customers who are at risk of leaving the platform, and helps companies understand the reasons for their departure. Furthermore, understanding the reasons for successful customer retention helps in generating higher customer lifetime value. For example, a company can improve its services or change marketing strategy by analyzing retention reasons (which, in turn, also helps to attract new customers).
Pricing Strategy
Machine learning algorithms are also used for pricing optimization. An optimal price is not calculated solely based on demand. An approach amounting to ‘when demand is high, prices are high, and when demand is low, prices are low’ is too simplistic for this context. In e-commerce, it is important to account for many factors at once: competitors’ prices, the time of day, a customer’s attitude, warehouse stock, and the season. Having such a nuanced approach will allow the company to have dynamic prices and thus win sales every time. For example, you can offer the best price for some products, or send coupons to certain customers in order to achieve customer tolerance. For some customers, this becomes an exciting game in which they can try to hunt for the best prices. Dynamic pricing brings significant results in the short term perspective: a 2-7% increase in business margins and a 200-350% average growth in ROI over the course of a year.
Chatbots
One of the cheaper options for improving customer service is using chatbots. Chatbots are based on AI. They use natural language processing (NLP) or speech recognition (in the case of phone calls), to interpret users’ questions and respond to them. They can communicate with a customer, identify an issue, and resolve it.
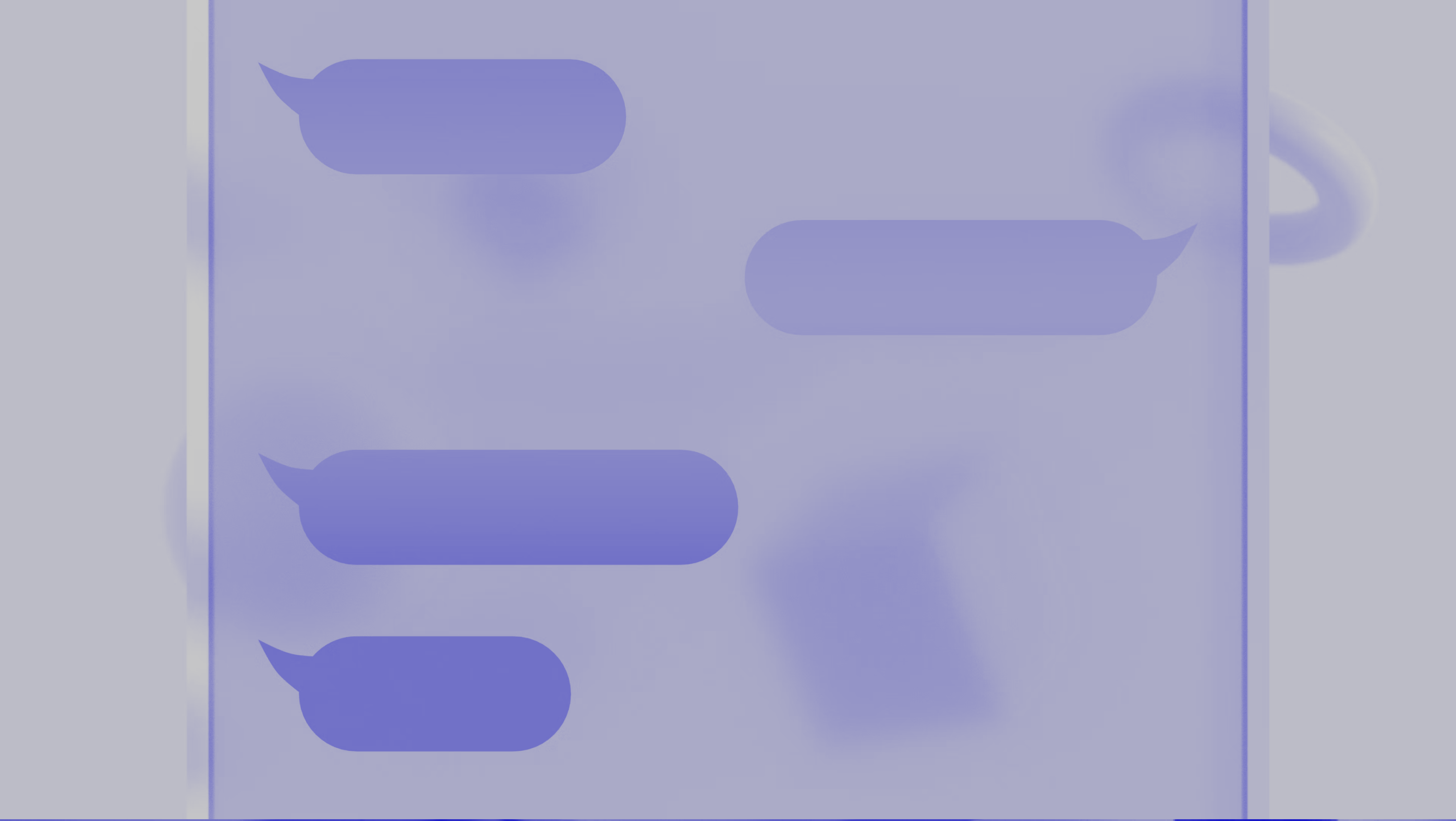
2 Data Science in E-Commerce
Customers may sometimes experience issues with site navigation; this can occur when they want to find a product page, FAQs, or sales, to name a few examples. Chatbots can help to find, choose and make an order of the product the customer is looking for. Also, chatbots carry out through transaction process. The advantages of the services offered are obvious:
· Answering questions 24/7
· Providing realistic conversation
· Giving higher satisfaction to customers
Fraud Detection
E-commerce is a field with high scammer activity caused by close connection to payments. There are many fraud schemes in e-commerce, like chargeback, unauthorized discounts, unauthorized sale voiding, returns, and many others. Thus, with all factors considered, AI offers the best solution for fraud prevention.
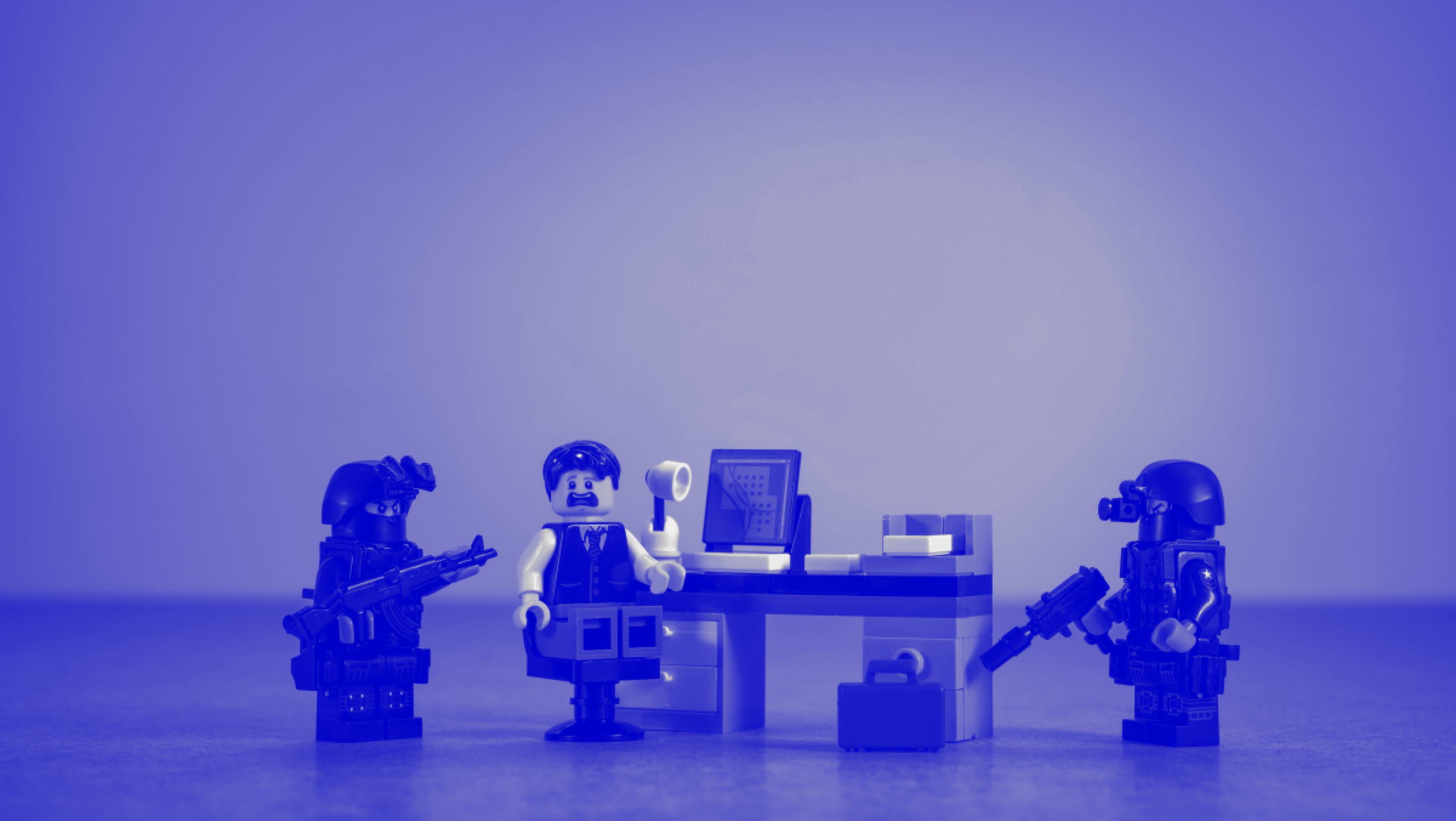
3 Data Science in E-Commerce
A fraud detection system collects and analyzes customers’ historical data in order to learn normal customer behavior, such as the devices they typically use, as well as the time and location of their activity. Abnormal account activity may indicate, for example, that the account or credit card of a customer was stolen. Also, AI can detect subtle behavioral patterns of scammers and identify persons that abuse the refund policy; this is a fairly well-known kind of scam, in which a fraudster orders a product, then returns a fake one.
Conclusion
Big data brings competitive advantages for e-commerce companies. Big e-commerce organizations like Amazon, eBay, and Netflix have long benefitted from the positive effects of using data science methods. Through data science, small companies find cheap solutions to increase their management effectiveness.
Interesting For You
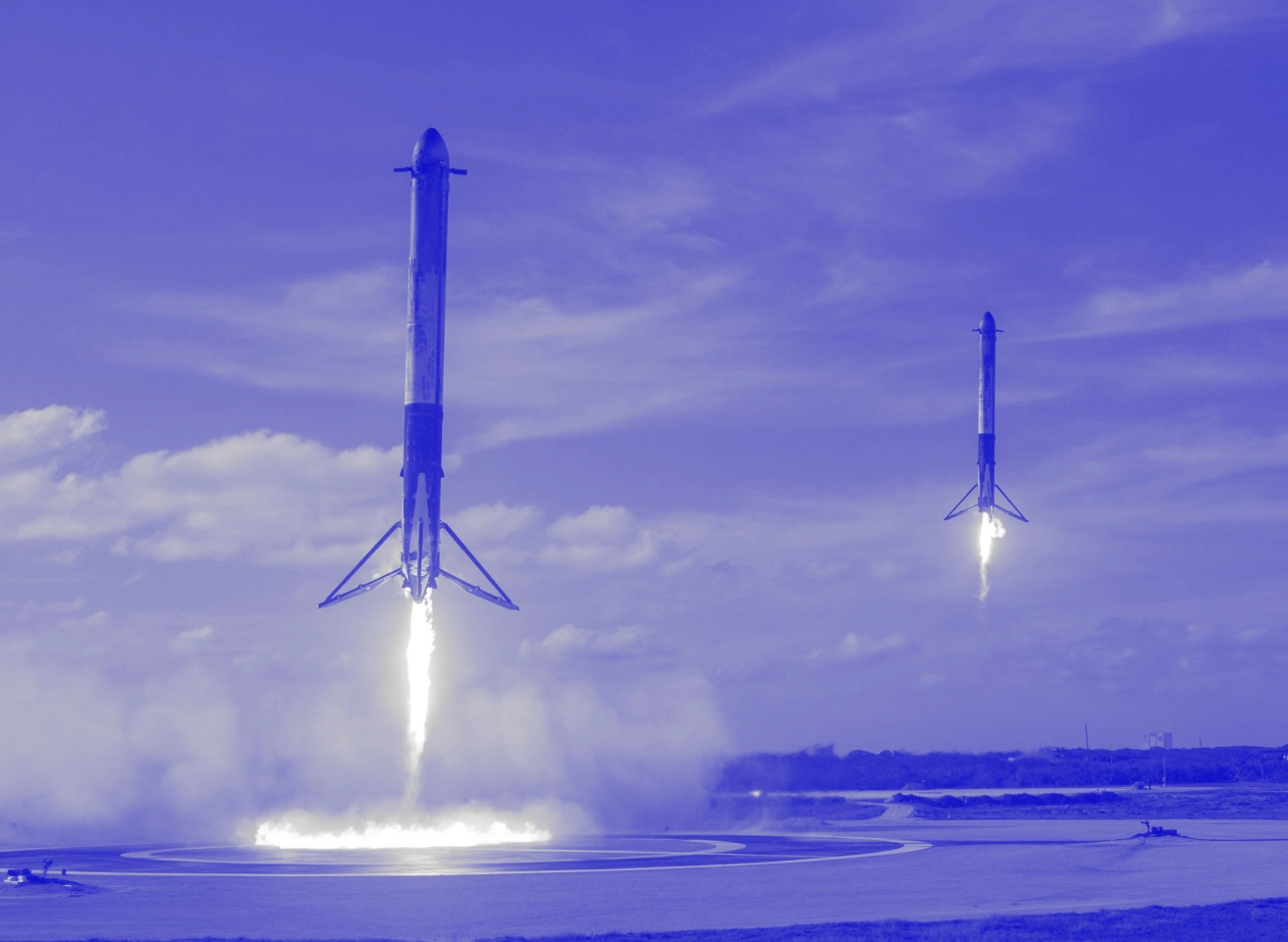
Predictive Analysis in Business
Decision-making in business is often based on assumptions about the future. Many companies aspire to develop and deploy an effective process for understanding trends and relationships in their activity in order to gain forward-looking insight to drive business decisions and actions. This is called predictive analytics. We can define predictive analytics as a process that uses data and a set of sophisticated analytic tools to develop models and estimations of an environment's behavior in the future. In predictive analysis, the first step is to collect data. Depending on your target, varied sources are using, such as web archives, transaction data, CRM data, customer service data, digital marketing and advertising data, demographic data, machine-generated data (for example, telemetric data or data from sensors), and geographical data, among other options. It is important to have accurate and up to date information. Most of the time, you will have information from multiple sources and, quite often, it will be in a raw state. Some of it will be structured in tables, while the rest will be semi-structured or even unstructured, like social media comments. The next important step is to clean and organize the data - this is called data preprocessing. Preprocessing usually takes up 80% of the time and effort involved in all analysis. After this stage, we produce a model using already existing tools for predictive analytics. It is important to note that we use collected data to validate the model. Such an approach is based on the main assumption of predictive analytics, which claims that patterns in the future will be similar to the ones in the past. You must ensure that your model makes business sense and deploy the analytics results into your production system, software programs or devices, web apps, and so on. The model can only be valid for a certain time period, since reality is not static and an environment can change significantly. For example, the preferences of customers may change so fast that previous expectations become outdated. So, it is important to monitor a model periodically. There are plenty of applications for business based on predictive analytics. To conclude this article, we will briefly consider some of them.
Read article
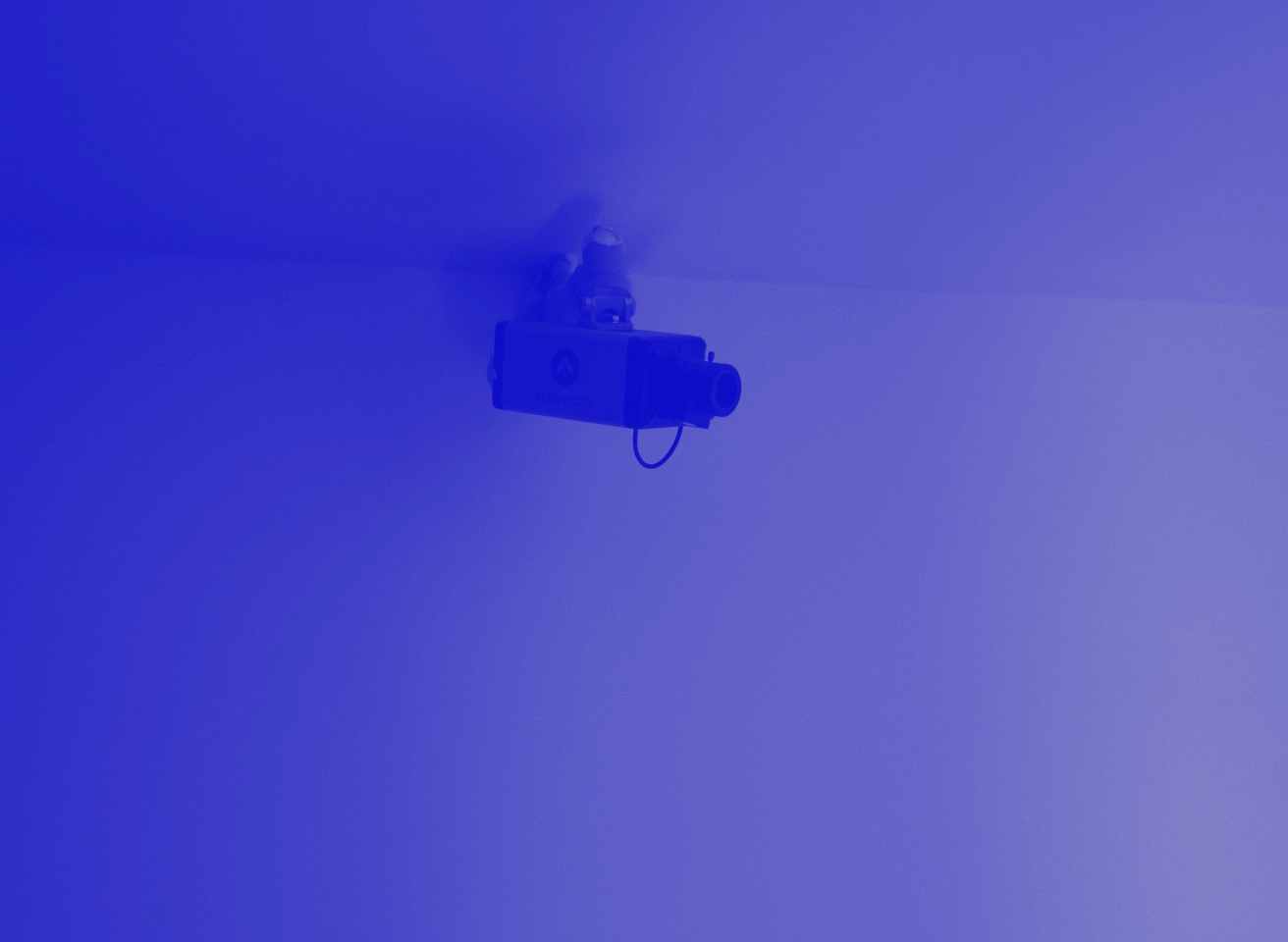
Fraud Detection
Fraud losses are the subject of constant interest by organizations and individuals alike. Interest in this area is justified, given that in 2018, 49% of organizations said they had been victims of fraud and economic crime according to PwC. Worldwide card fraud losses totalled $24.26 billion in 2017 according to The Nilson Report. Fraud is a widespread, global issue. Organizations should always monitor their data in order to be fraud resistant. The automatization of this process can reduce costs and detect fraud faster. A powerful helper in fraud detection and understanding how fraud works is Data Science. In addition to detecting known types of fraud, data analysis techniques help to uncover new types of fraud.
Read article
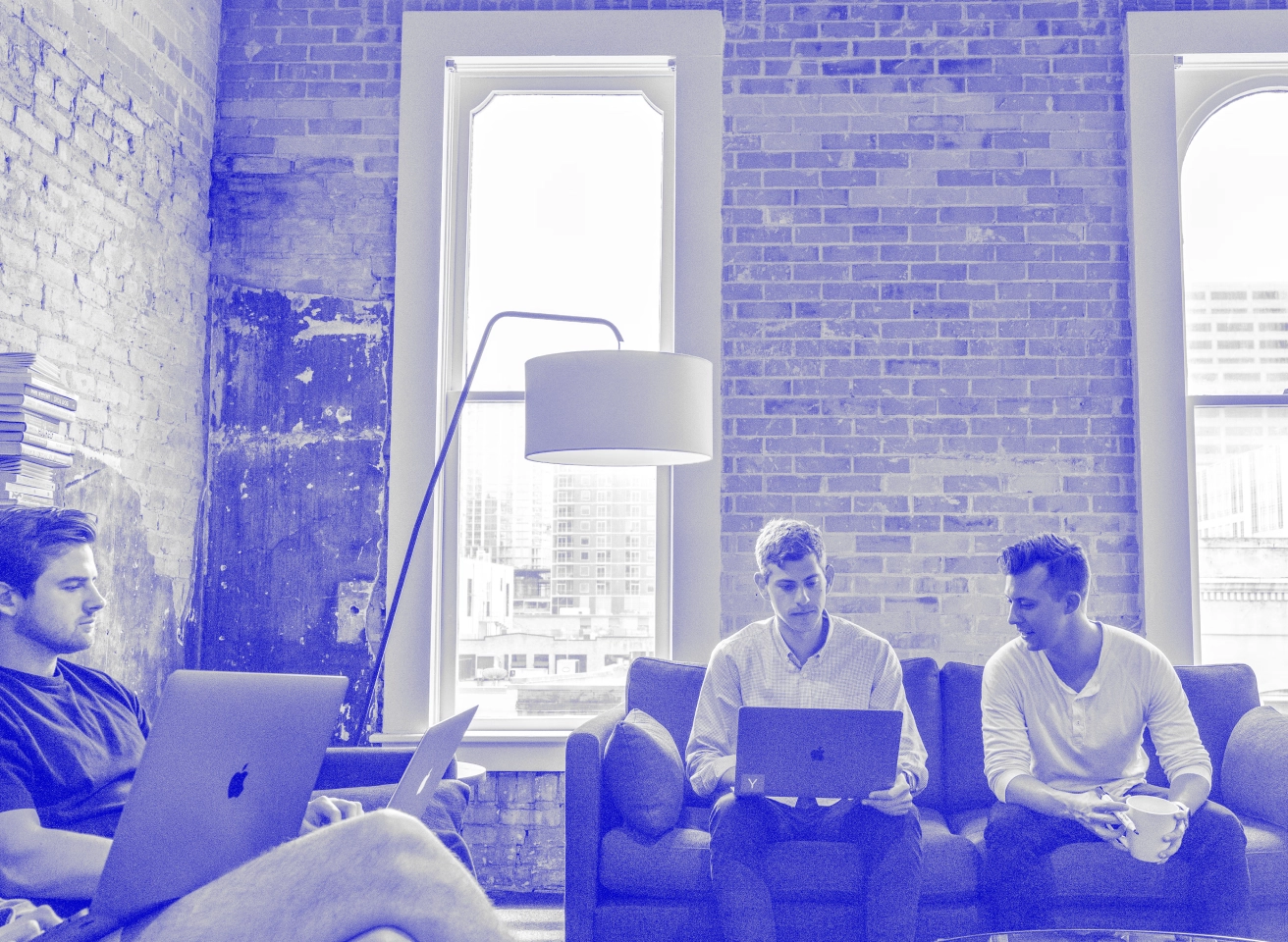
Data Science in Human Resources
Do companies need to use Data science when hiring new employees? Big data has changed the requirement process, and most organizations’ activities more broadly. The scientific analysis era has touched the human resources sector too. Effective data science techniques can provide better quality, higher accuracy, and a cost-effective outcome for HR. Let’s see how data science techniques can help with different fields and work phases of HR.
Read article