Data Science in Human Resources
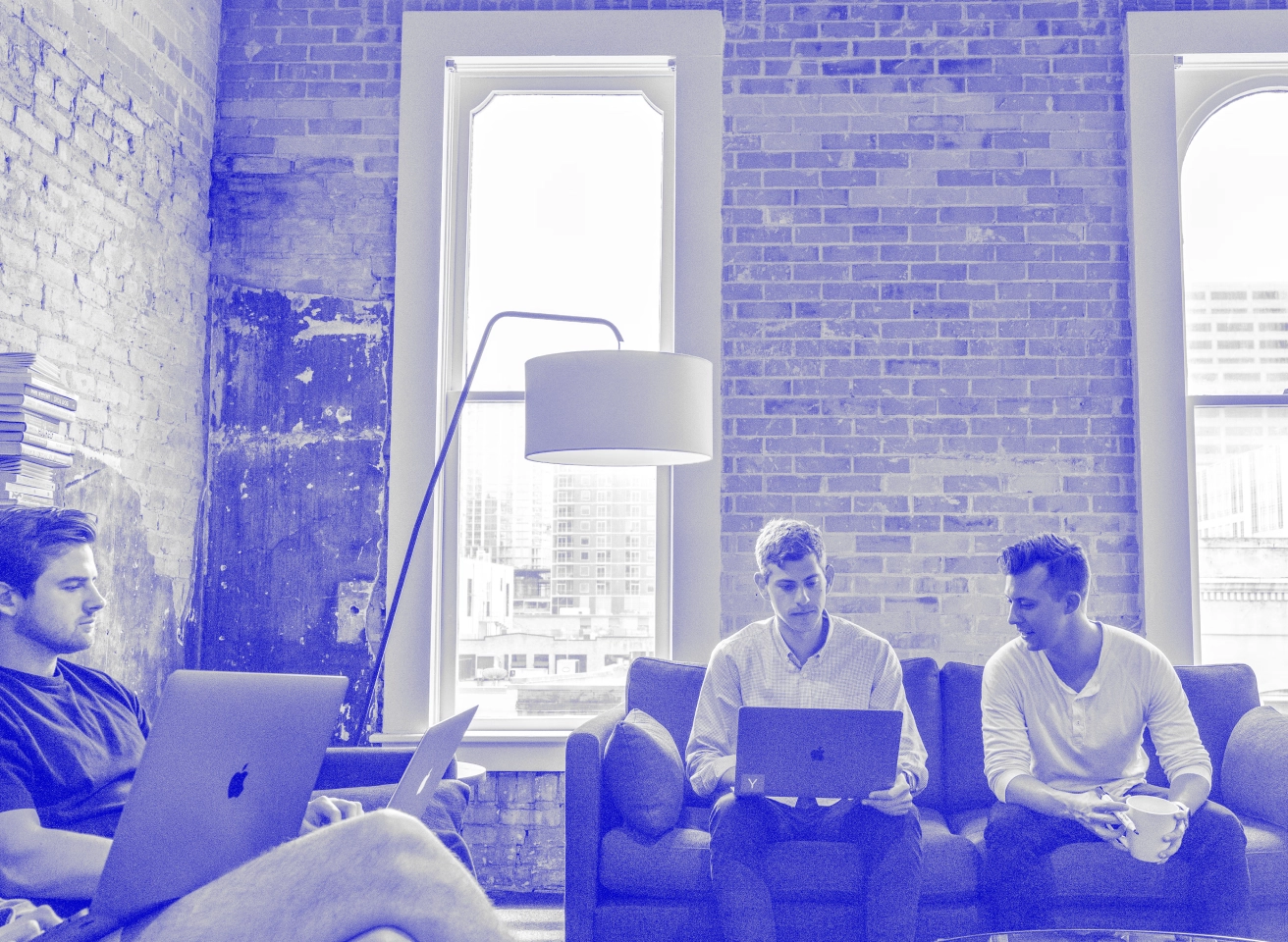
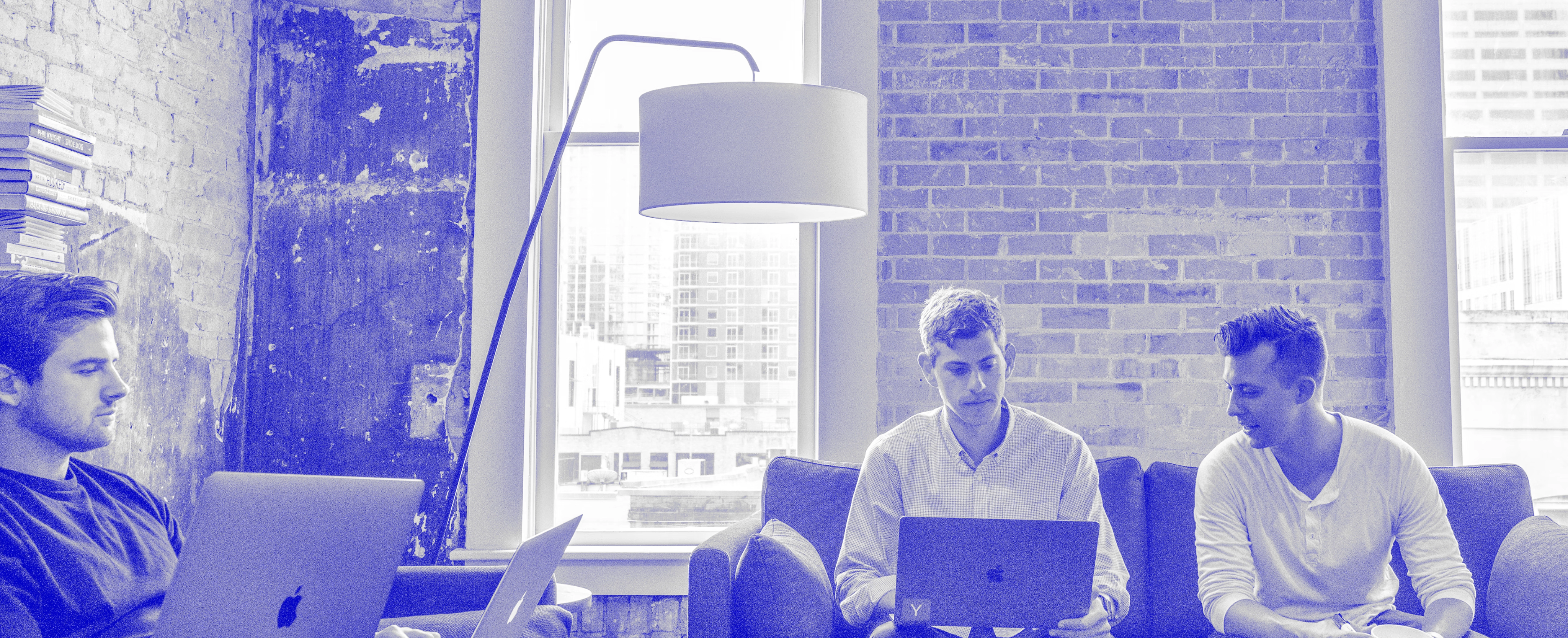
Do companies need to use Data science when hiring new employees? Big data has changed the requirement process, and most organizations’ activities more broadly. The scientific analysis era has touched the human resources sector too. Effective data science techniques can provide better quality, higher accuracy, and a cost-effective outcome for HR. Let’s see how data science techniques can help with different fields and work phases of HR.
Hiring
Internet technologies and the increasing mobility of people have made the hiring process very competitive. Companies spend an average of 23.7 days on hiring a new employee, while the best candidates stay on the market for only ten days. On the other hand, many companies avoid hiring from popular job websites. Posting a vacancy on LinkedIn or Indeed may bring in thousands of resumes from candidates. Of these applicants, most are not sufficiently qualified, especially for technical positions. So, HR teams have three important goals to meet during the hiring phase: to make the hiring process faster, to attract more talent, and to analyze more resumes.
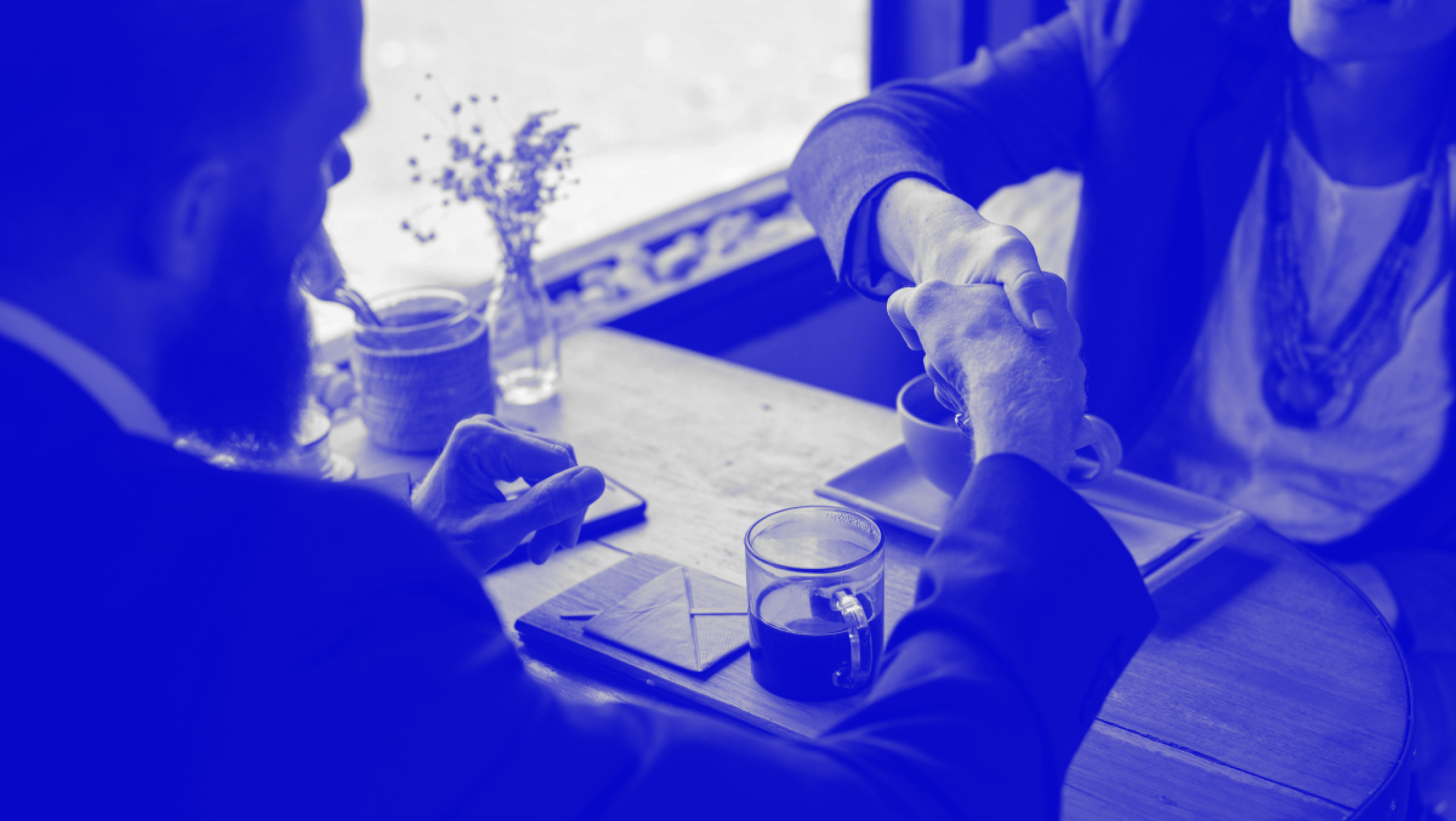
1 Data Science in Human Resources
Predictive analytics technologies and natural language processing help HR teams to filter through thousands of resumes, focus on the best channels for hiring and create a base of the most promising candidates on a specific market. The main advantages of using data science in hiring are (a) a decrease in hiring time and (b) an increase in candidate quality for the company. Predictive hiring in the recruitment process is successfully used by big companies. For example, the implementation of a predictive hiring tool in Wells Fargo increased retention rates for tellers by 15%.
Engagement and Performance
Predictive analysis can increase employee engagement and productivity. Even if a company has successfully gained a good employee, this is only the beginning of a longer process. The candidate should be introduced to their new duties and involved in professional training programs. Many companies have realized that the cost of training is higher than the profit they receive from it.
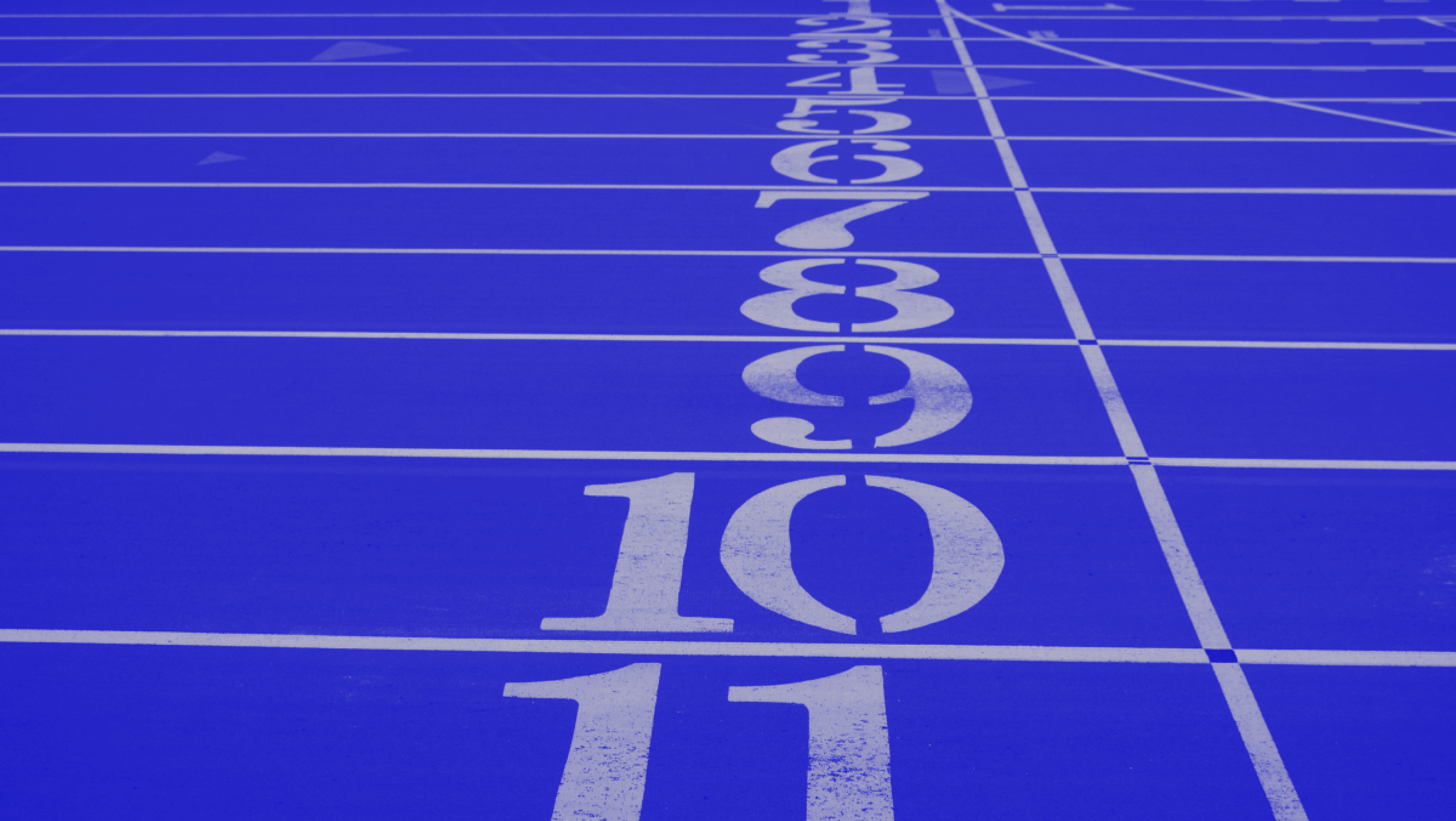
3 Data Science in Human Resources
Data science can examine employees’ learning levels and help to modify training courses, in order to make them more productive. Using Big data analysis, companies can also detect potential mistakes and flaws in work. For example, JetBlue wanted to improve on training attrition with new members joining the team. To accomplish this, JetBlue used data science methods in their analysis. As a result, training failure-based attrition fell by 75%, and overall training attrition fell by 25%.
Retention
One of the biggest challenges companies face is staff retention. In general, companies invest money and resources in hiring new people, and then allocate 1-3 months to training new employees. This investment will be a loss if a person decides to leave within their first six months of employment. Employees may become dissatisfied due to a variety of reasons: low salary and poor communication with superiors are just two examples of many impacting factors. Employers can never be sure that the members of a “strong team ” will be staying at the company for the foreseeable future. One third of employees at companies with 100 plus employees are currently looking for jobs. Predictive analysis can find the hidden reasons for employee dissatisfaction and allow HR management to find solutions before the main problems come to a head. Machine learning techniques scan employees’ attributes to detect increases or decreases in resignations

4 Data Science in Human Resources
Besides retention, there is also employee turnover. This term refers to the percentage of workers who leave an organization and are replaced by new employees. There are many factors which affect turnover in a particular organization. Some of them can be easily resolved, but some present more of a challenge. In the second case, predictive analytics uses historical data to predict how many employees will leave the company and when they will do so. Thus, the HR team can start looking for new candidates before old employees resign.
Conclusion
Finally, it is worth noting that big data analytics have become the new force for contemporary HR management. New data science approaches decrease the time and resources required to hire better workers. These approaches also increase employee engagement, their productivity, and retention rates. While the human factor in HR work is very important, the implementation of machine learning techniques in HR teams increases their productivity and eliminates routine.
Interesting For You
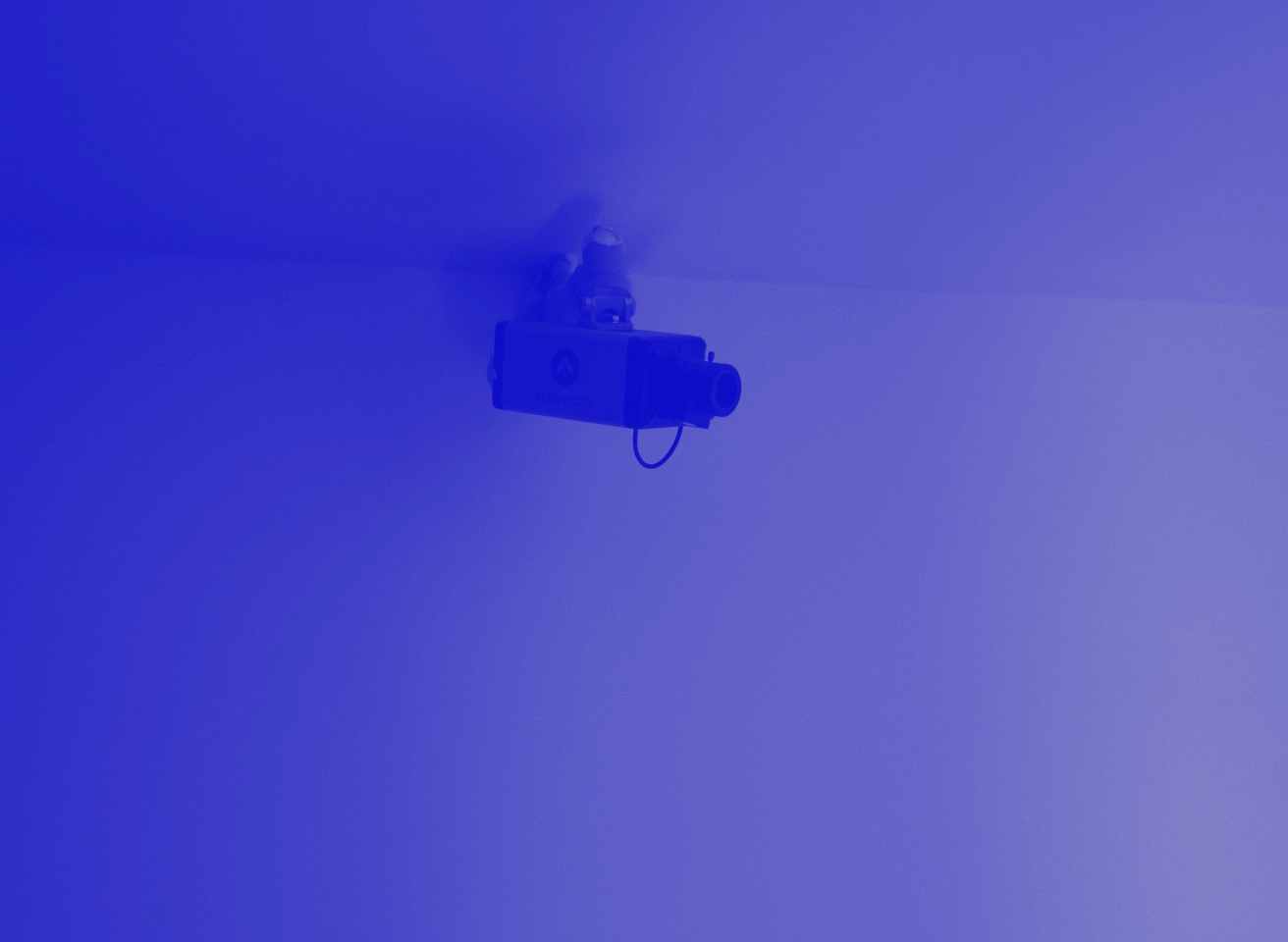
Fraud Detection
Fraud losses are the subject of constant interest by organizations and individuals alike. Interest in this area is justified, given that in 2018, 49% of organizations said they had been victims of fraud and economic crime according to PwC. Worldwide card fraud losses totalled $24.26 billion in 2017 according to The Nilson Report. Fraud is a widespread, global issue. Organizations should always monitor their data in order to be fraud resistant. The automatization of this process can reduce costs and detect fraud faster. A powerful helper in fraud detection and understanding how fraud works is Data Science. In addition to detecting known types of fraud, data analysis techniques help to uncover new types of fraud.
Read article
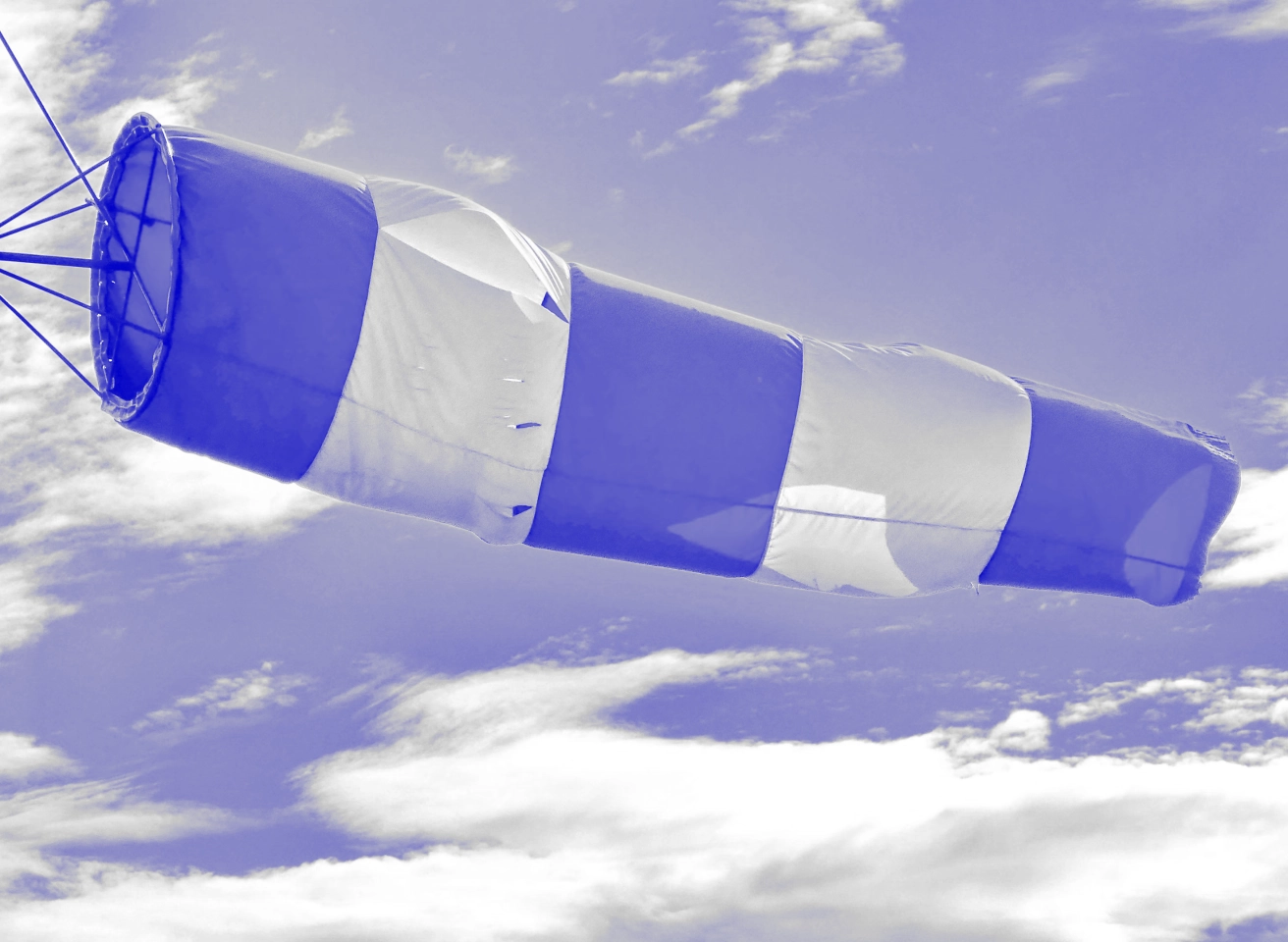
Predictive Analytics Workflow
Many companies use predictive models in their activity to provide better customer service, sell more products and services to customers, manage risk from fraudulent activity, and better plan the use of their human resources (to list a few important examples). How does predictive analysis offer all of these benefits? In this article we will consider the process of predictive analytics, and its related advantages.
Read article
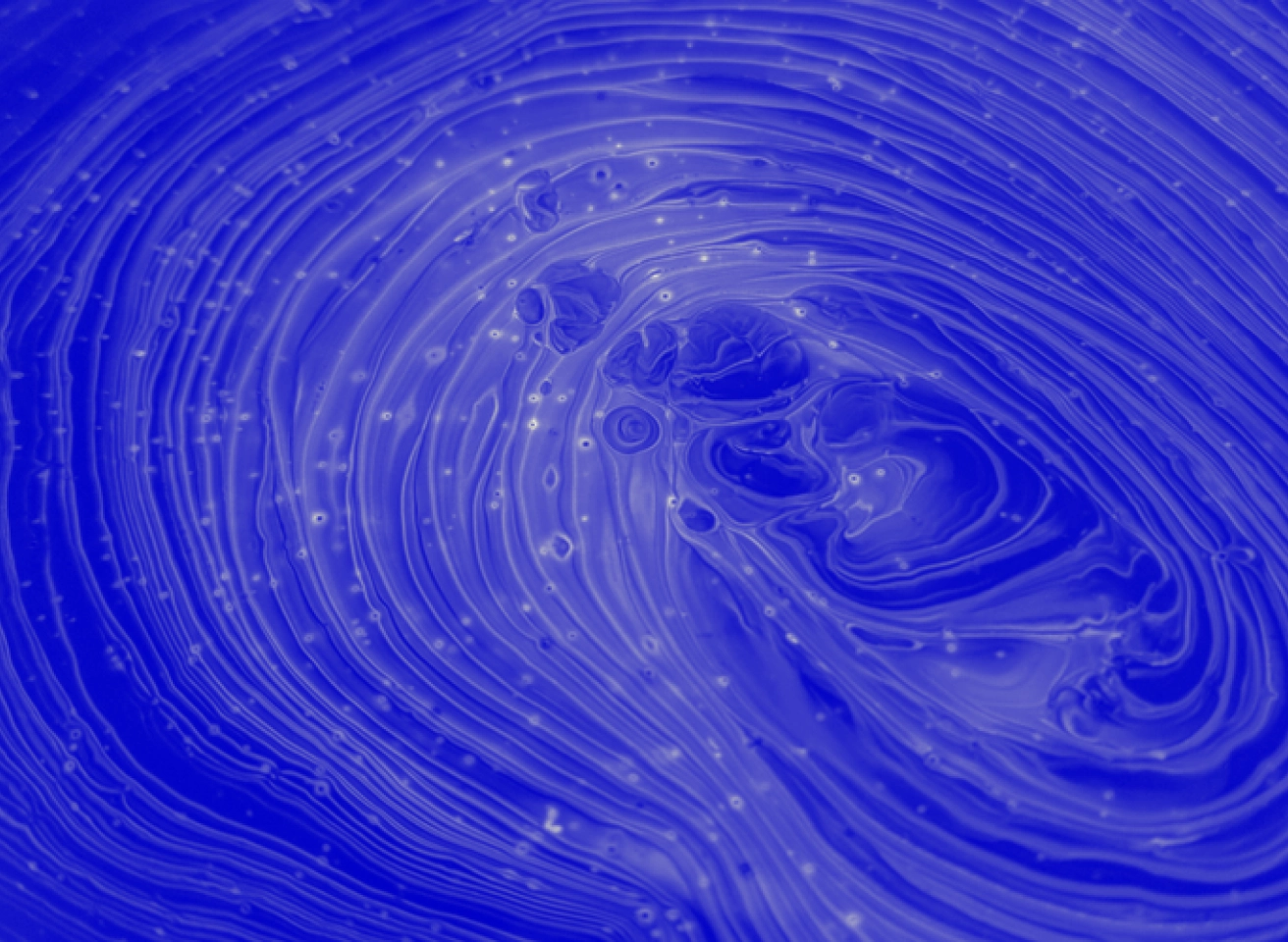
What is Data Science?
In recent years, data science has become increasingly prominent in the common consciousness. Since 2010, its popularity as a field has exploded. Between 2010 and 2012, the number of data scientist job postings increased by 15 000%. In terms of education, there are now academic programs that train specialists in data science. You can even complete a PhD degree in this field of study. Dozens of conferences are held annually on the topics of data science, big data and AI. There are several contributing factors to the growing level of interest in this field, namely: 1. The need to analyze a growing volume of data collected by corporations and governments 2. Price reductions in computational hardware 3. Improvements in computational software 4. The emergence of new data science methods. With the increasing popularity of social networks, online services discovered the unlimited potential for monetization to be unlocked through (a) developing new products and (b) having greater information and data insights than their competitors. Big companies started to form teams of people responsible for analyzing collected data.
Read article