Fraud Detection
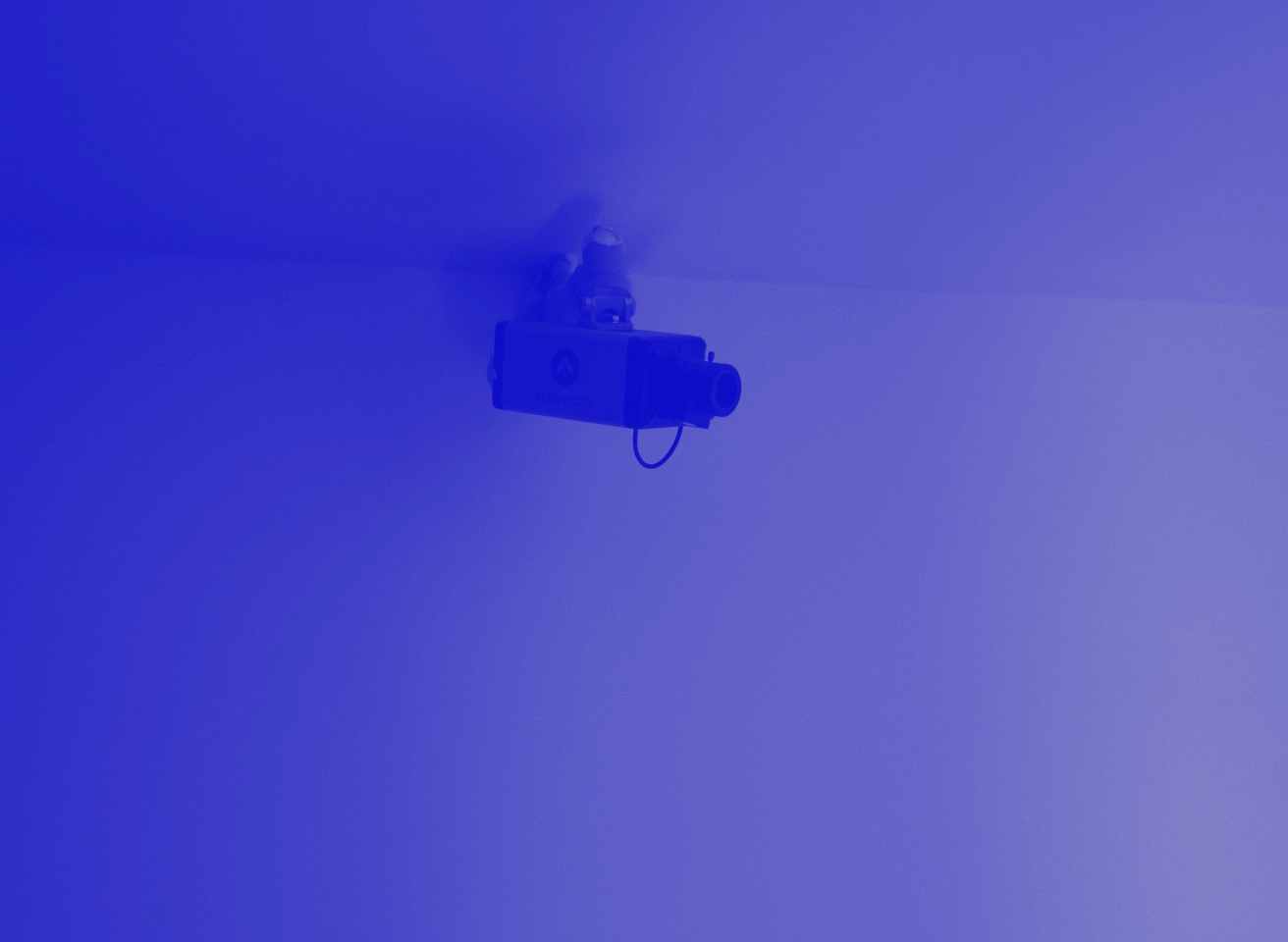
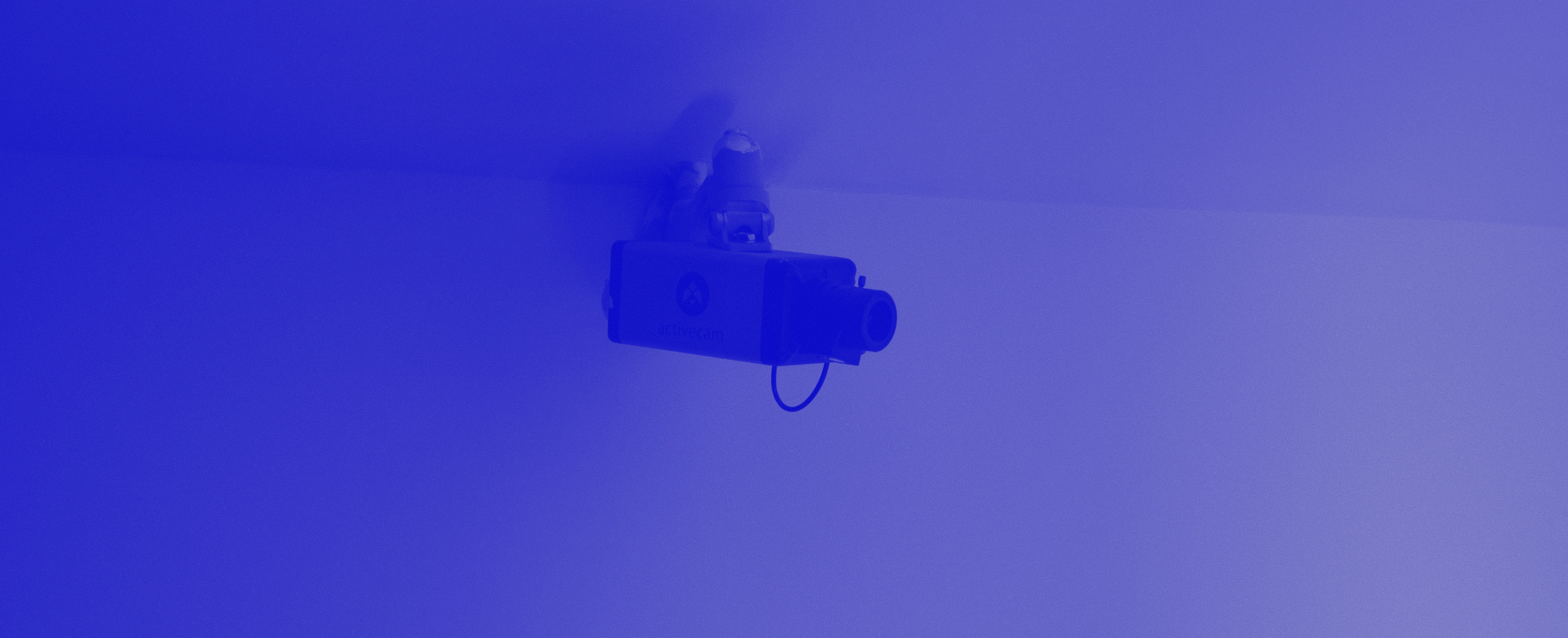
Fraud losses are the subject of constant interest by organizations and individuals alike. Interest in this area is justified, given that in 2018, 49% of organizations said they had been victims of fraud and economic crime according to PwC. Worldwide card fraud losses totalled $24.26 billion in 2017 according to The Nilson Report. Fraud is a widespread, global issue. Organizations should always monitor their data in order to be fraud resistant. The automatization of this process can reduce costs and detect fraud faster. A powerful helper in fraud detection and understanding how fraud works is Data Science. In addition to detecting known types of fraud, data analysis techniques help to uncover new types of fraud.
Banking
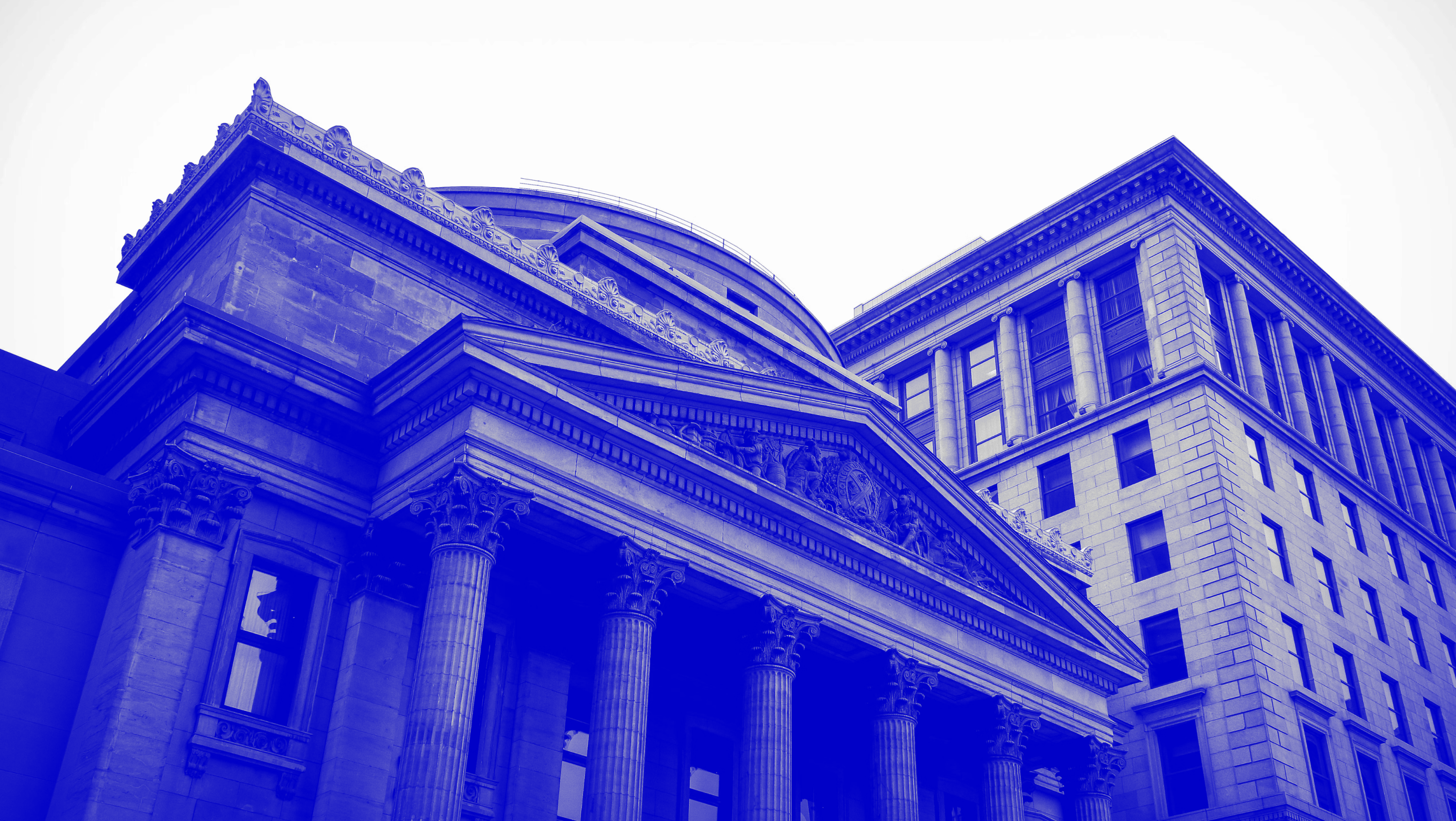
1 Fraud Detection
For a huge number of online transactions in banking, human analysis is not able to detect fraud. Another circumstance requiring the usage of Data Science techniques is a need to detect fraudulent transactions in real time. The implementation of real time analysis of the potential risks allows companies or individuals to take proactive measures.
When it comes to detecting anomalies in transactions, the main challenge lies in recognizing their origins; anomalies may emerge due to fraud, but they may also be caused by errors or missing data. Another complexity in machine analysis of fraud is highly imbalanced classes. For two-class cases, frauds and ordinary transactions, the majority class is the negative class - ordinary transactions - and the minority or rare class is the positive class - frauds. The minority class is very infrequent, approximately 1% of the whole dataset, and traditional classifiers are likely to predict everything as the majority class, which gives a “high precision” of about 99%. Examples of such frauds in banking with imbalanced classes include money laundering, terrorist financing, credit card fraud, identity fraud perpetrated through customer account takeover, synthetic identities, nefarious applications and other financial crimes. Besides identifying true positives, financial companies need to avoid false positive fraud detection. A high false positive rate requires more human resources for checking all cases with a fraud flag and, consequently, leads to poor customer service.
Here are some examples of fraud prevention systems in banking. AI algorithms detect unusual high transactions and put them on hold until the customer confirms the deal. Fraud detection algorithms investigate and block multiple accounts opened in a short period with similar data. Using data analytics, banks use geotiming methods to compare the geographical locations of in-person card swipes with the amount of time elapsed between them. If the time taken between two swipes is not enough for the customer to travel between them, this activity will be flagged as a fraud.
An example of efficient modernization of the fraud detection process with Deep Learning is Danske Bank. This bank had a low 40% fraud detection rate, and 99.5% of false positive cases the bank was investigating were not fraud related. The implementation of a modern analytic solution leveraging AI allowed the bank to realize a 60% reduction in false positives, increase true positives by 50%, and focus human resources on actual cases of fraud
Insurance
A major challenge that insurance companies face in detecting fraud claims is dealing with a large number of documents supporting claims, like witness reports, police reports, and claim forms. Each claim requires manual processing, in order to recognize misrepresented facts on an insurance application, inflated actual claims, claims for injuries or damage that never occurred (in the event of staging accidents and so on).
Insurers enrich their internal data, such as call center notes, voice recordings, with third party details on weather, traffic, news feeds, clients’ social media data, bills, wages, criminal records, address changes, etc. Then, using Data Science tools, they analyze across multiple data sets to gain insight on potentially fraudulent claims. For example, algorithms detect that one person arises as a witness multiple times or in multiple positions, as a pedestrian, driver, and/or opponent of the accident. In these cases, all the claims will be flagged as frauds.
Telecommunications
The telecommunication industry attracts a significant number of users every day and cannot afford to avoid using Data Science. The common examples of fraud in the telecom field include the following: illegal access and authorization, theft and fake profiles, behavioral fraud, etc. Machine Learning algorithms can identify anomalies in the normal traffic of a user and prevent fraud. Some mobile operators share cell phone GPS data with banks, which helps to verify a person’s card use against their location and, thus, prevent credit card fraud.
E-commerce
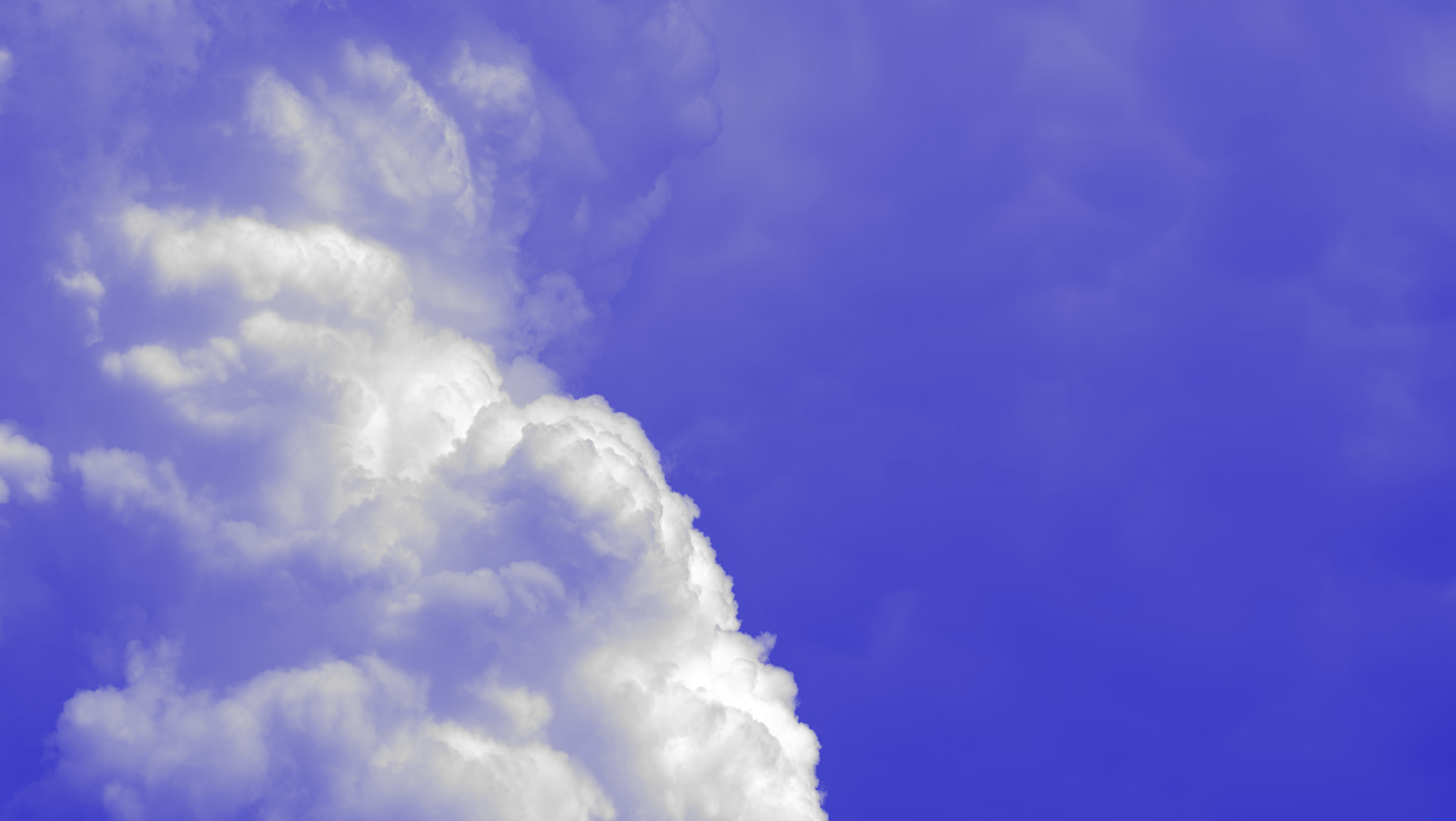
2 Fraud Detection
E-commerce is also a field with high scammer activity caused by close connection to payments. There are many fraud schemes in e-commerce, like chargeback, unauthorized discounts, unauthorized sale voiding, returns, and many others. Thus, AI offers the best solution for preventing fraud. Fraud detection systems collect and analyze the historical data of customers in order to learn normal customer behavior; this includes data on the devices used by a typical customer, the time, and the location. Abnormal account activity may indicate, for example, that the account or credit card of a specific customer was stolen. Also, AI can detect the subtle behavioral patterns of scammers and identify persons who abuse the refund policy. A good example of this includes a well-known scam, in which a fraudster orders a product and then returns a fake one.
Conclusion
The use of Data Science in fraud detection is not limited to the fields described above. It is already used in transportation, oil & gas, automotive industries, casinos, hotels, and the higher education system.
Interesting For You
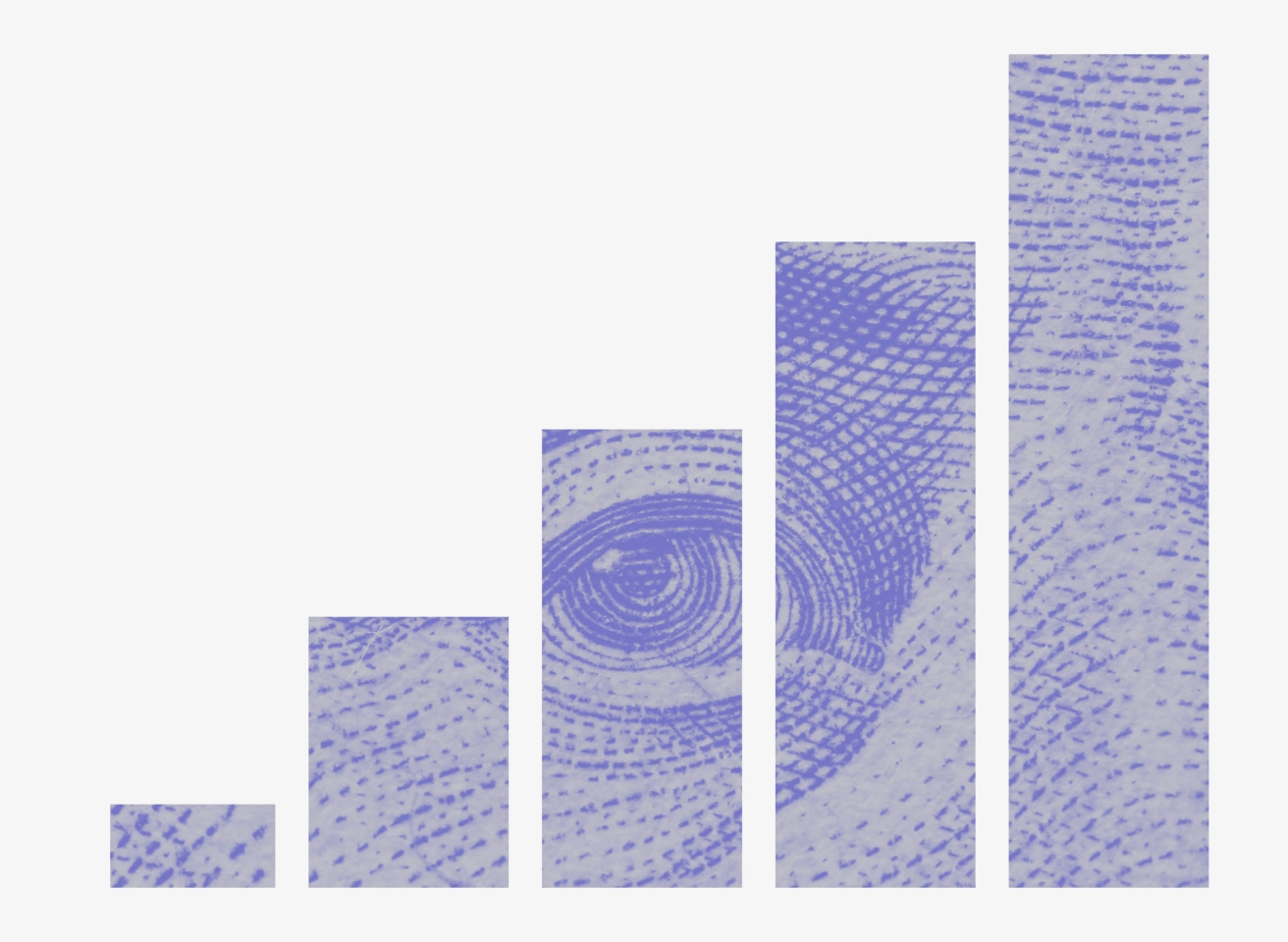
Data Science in E-Commerce
More than 20 years ago, e-commerce was just a novel concept, until Amazon sold their very first book in 1995. Nowadays, the e-commerce market is a significant part of the world’s economy. The revenue and retail worldwide expectations of e-commerce in 2019 were $2.03 trillion and $3.5 trillion respectively. This market is developed and diverse both geographically and in terms of business models. In 2018, the two biggest e-commerce markets were China and the United States, with revenues of $636.1 billion and $504.6 billion respectively. Currently, the Asia-Pacific region shows a better growth tendency for e-commerce retail in relation to the rest of the world. Companies use various types of e-commerce in their business models: Business-to-Business (B2B), Business-to-Consumer (B2C), Consumer-to-Consumer (C2C), Consumer-to-Business (C2B), Business-to-Government (B2G), and others. This diversity has emerged because e-commerce platforms provide ready-made connections between buyers and sellers. This is also the reason that B2B’s global online sales dominate B2C: $10.6 trillion to $2.8 trillion. Rapid development of e-commerce generates high competition. Therefore, it’s important to follow major trends in order to drive business sales and create a more personalized customer experience. While using big data analytics may seem like a current trend, for many companies, data science techniques have already been customary tools of doing business for some time. There are several reasons for the efficiency of big data analytics: · Large datasets make it easier to apply data analytics; · The high computational power of modern machines even allows data-driven decisions to be made in real time; · Methods in the field of data science have been well-developed. This article will illustrate the impact of using data science in e-commerce and the importance of data collection, starting from the initial stage of your business.
Read article
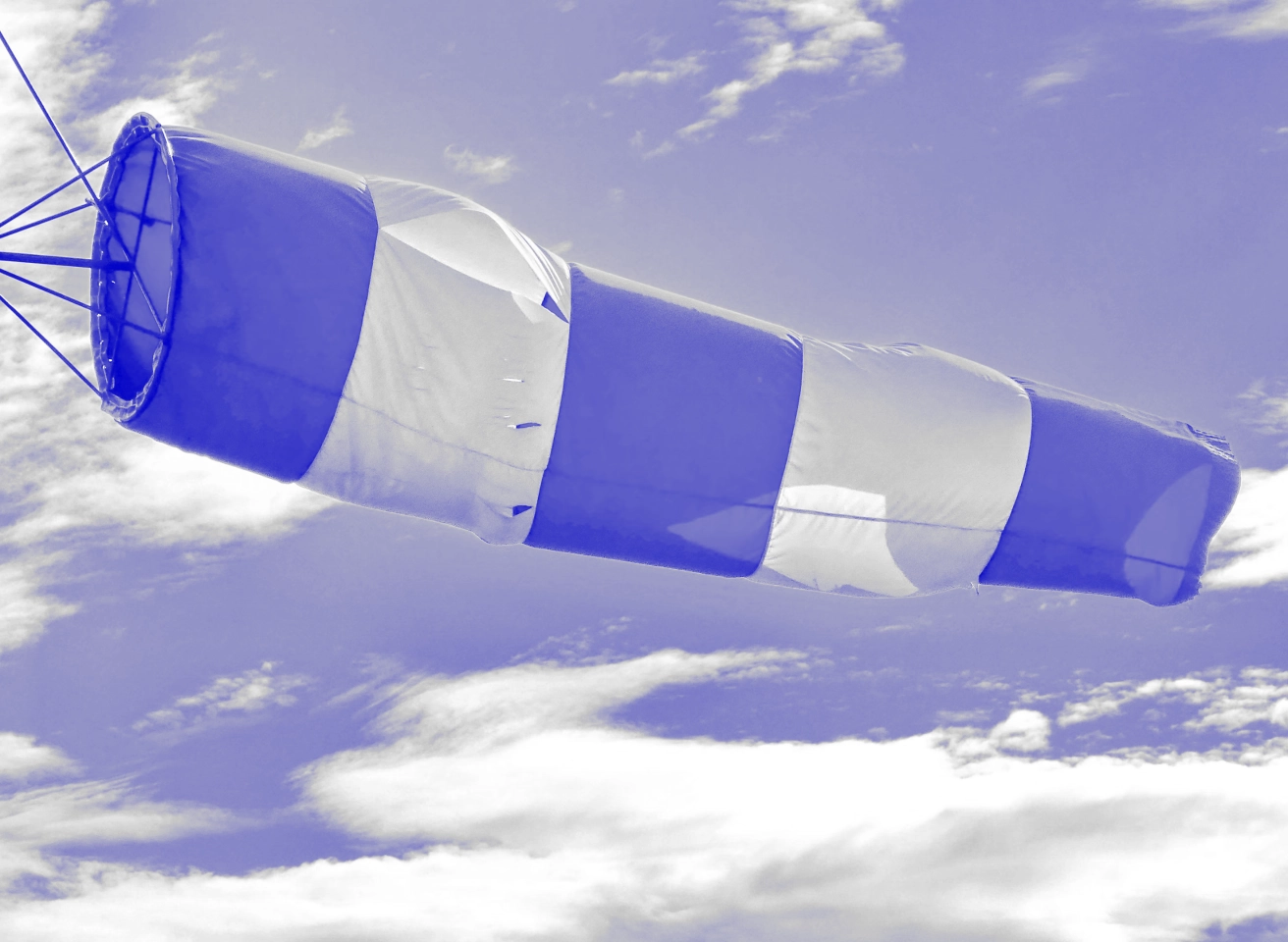
Predictive Analytics Workflow
Many companies use predictive models in their activity to provide better customer service, sell more products and services to customers, manage risk from fraudulent activity, and better plan the use of their human resources (to list a few important examples). How does predictive analysis offer all of these benefits? In this article we will consider the process of predictive analytics, and its related advantages.
Read article
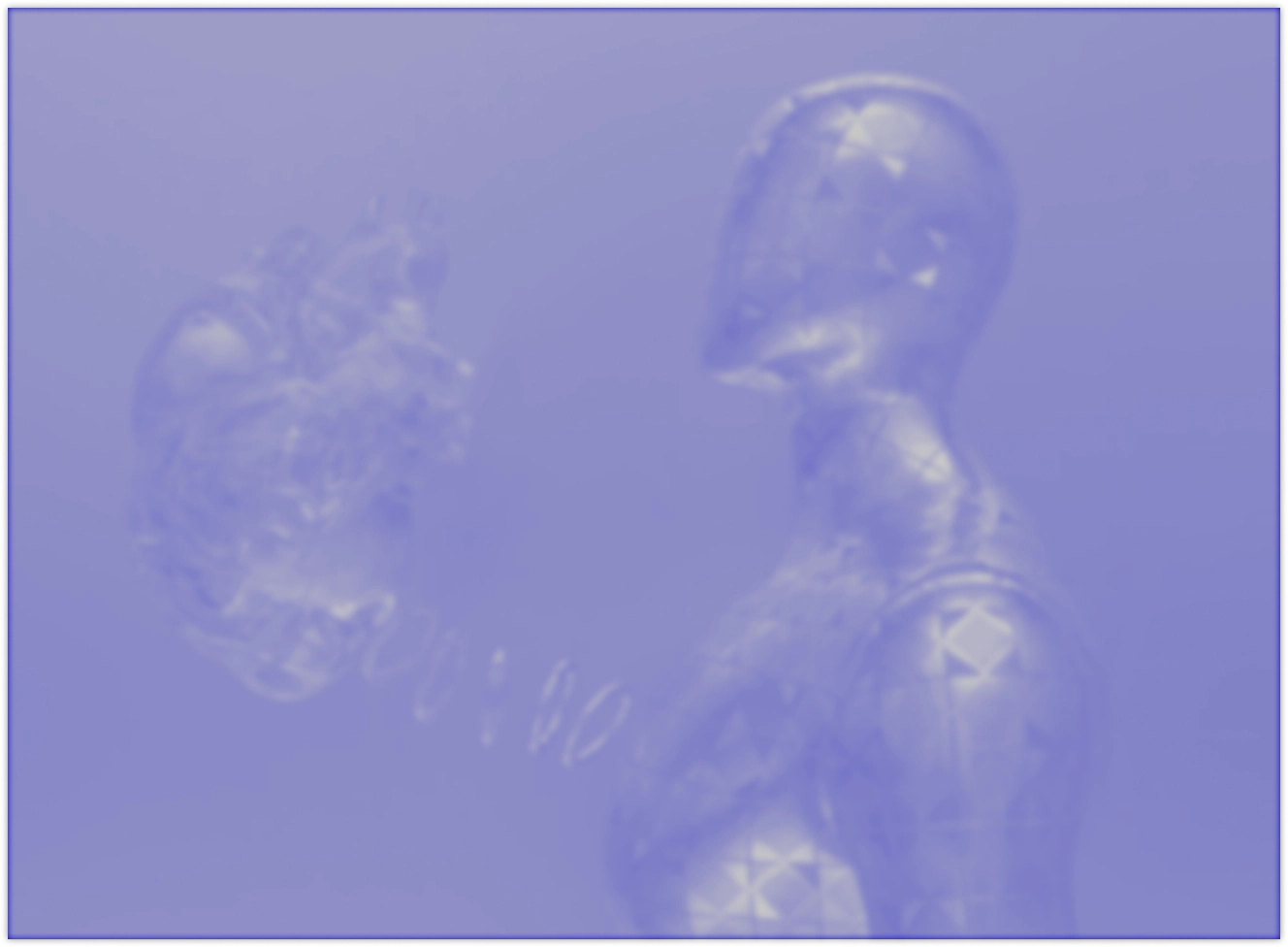
Sentiment Analysis in NLP
Sentiment analysis has become a new trend in social media monitoring, brand monitoring, product analytics, and market research. Like most areas that use artificial intelligence, sentiment analysis (also known as Opinion Mining) is an interdisciplinary field spanning computer science, psychology, social sciences, linguistics, and cognitive science. The goal of sentiment analysis is to identify and extract attitudes, emotions, and opinions from a text. In other words, sentiment analysis is a mining of subjective impressions, but not facts, from users’ tweets, reviews, posts, etc.
Read article